Campus in the top three at the UN PET Lab hackathon
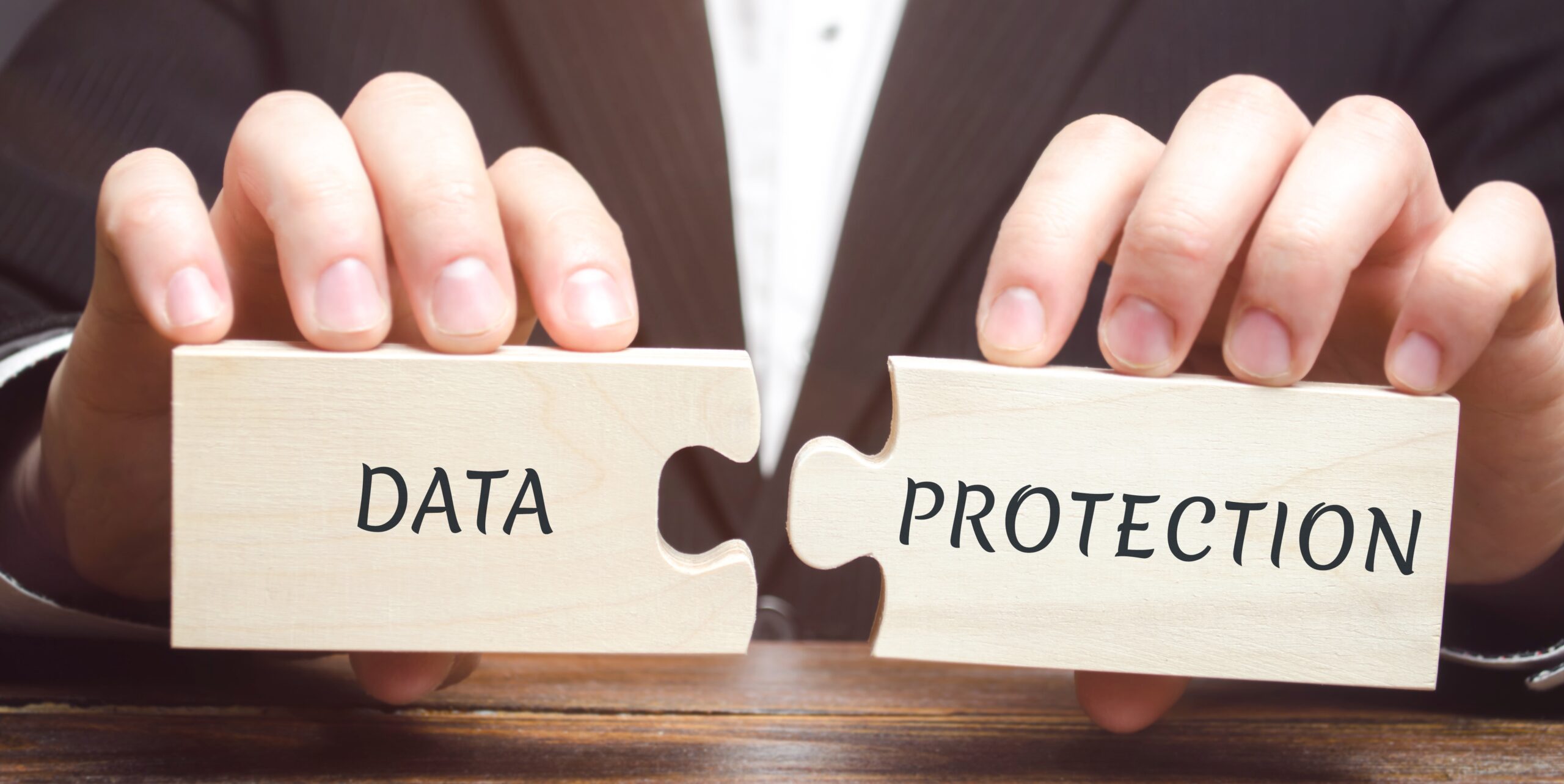
In November, three data scientists from the Office for National Statistics (ONS) Data Science Campus took part in the Privacy Enhancing Technologies (PETs) hackathon run alongside the International Conference on Big Data hosted in Yogyakarta, Indonesia.
Competing remotely, Michaela Lawrence, Mat Weldon and Henry Wilde went up against nearly 200 international teams, including representatives from other national statistical organisations (NSOs), data science start-ups and academic research centres. Our team battled through to achieve an impressive third place, closely behind the first-placed Oxford University research team and a Canadian PETs consultancy in second.
About the event
The event was organised by the UN PET Lab, a collection of NSOs and technology experts collaborating to modernise the way data are shared and statistics are produced. PETs allow safe data sharing and collaboration across institutions and national borders, allowing organisations to benefit from enhanced data access, without compromising on data privacy.
PETs consist of many different techniques, including encryption, noise addition and methods that enable analysts to train machine-learning models without having direct access to data.
The challenge
The competition was devised to increase awareness of PETs and their potential for use by organisations to allow data access for tackling important societal and economic questions.
It focused on survey data provided by the UN Refugee Agency (UNHCR) with detailed information about the refugee population in Kenya since the beginning of the coronavirus (COVID-19) pandemic. This is a sensitive dataset at household level, containing around 50 variables with detailed information of household composition, circumstances and living conditions.
Teams were tasked with accurately predicting three sensitive variables in a “test” subset of the data, while only being able to make noisy queries on another subset of the data. The teams were not able to directly view the sensitive variables, but were able to interact with them through methods that are known to preserve privacy. This included making noisy queries and creating synthetic data.
Differential privacy
Each interaction with the data had a cost. The magnitude of this cost was determined by how much noise the teams were willing to have added to their queries – the noisier the query, the cheaper it was. Noise was added using a method called differential privacy, which provides a formal mathematical definition of disclosure risk.
For example, to retrieve an estimate of the number of male and female respondents in the data, a random number centred on zero would be added to each count, in such a way that the ability of a snooper to learn about any individual in the data would be limited, no matter what other information they possessed. Adding more noise gives a stronger guarantee, whereas adding less noise (a random number closer to zero) gives a weaker guarantee and has a higher privacy cost. When there are many male and female respondents, this additional noise may not have a large impact on the accuracy of the estimate, but if we wanted to count many small categories, or occurrences of a rare event, the same amount of noise would have a bigger impact on accuracy.
Our approach
Final scores were determined by a trade-off between the accuracy of the predictions and the total cost of all the queries a team made. To finish third, our team used privacy-preserving versions of various methods to explore the data and improve its predictions, including data visualisation, principal components analysis, synthetic data and random forests.
The hackathon brought together an international community of data scientists and introduced some of the tools and frameworks on offer to implement PETs, demonstrating how these can allow data analysis without sharing sensitive microdata. The insights gained will inform our ongoing work to explore how we can use PETs to enable more integrated analyses of linked data across the public sector, to improve decision-making without compromising citizens’ privacy.