Use of hybrid data to understand the community-level influences on coronavirus (COVID-19) incidence
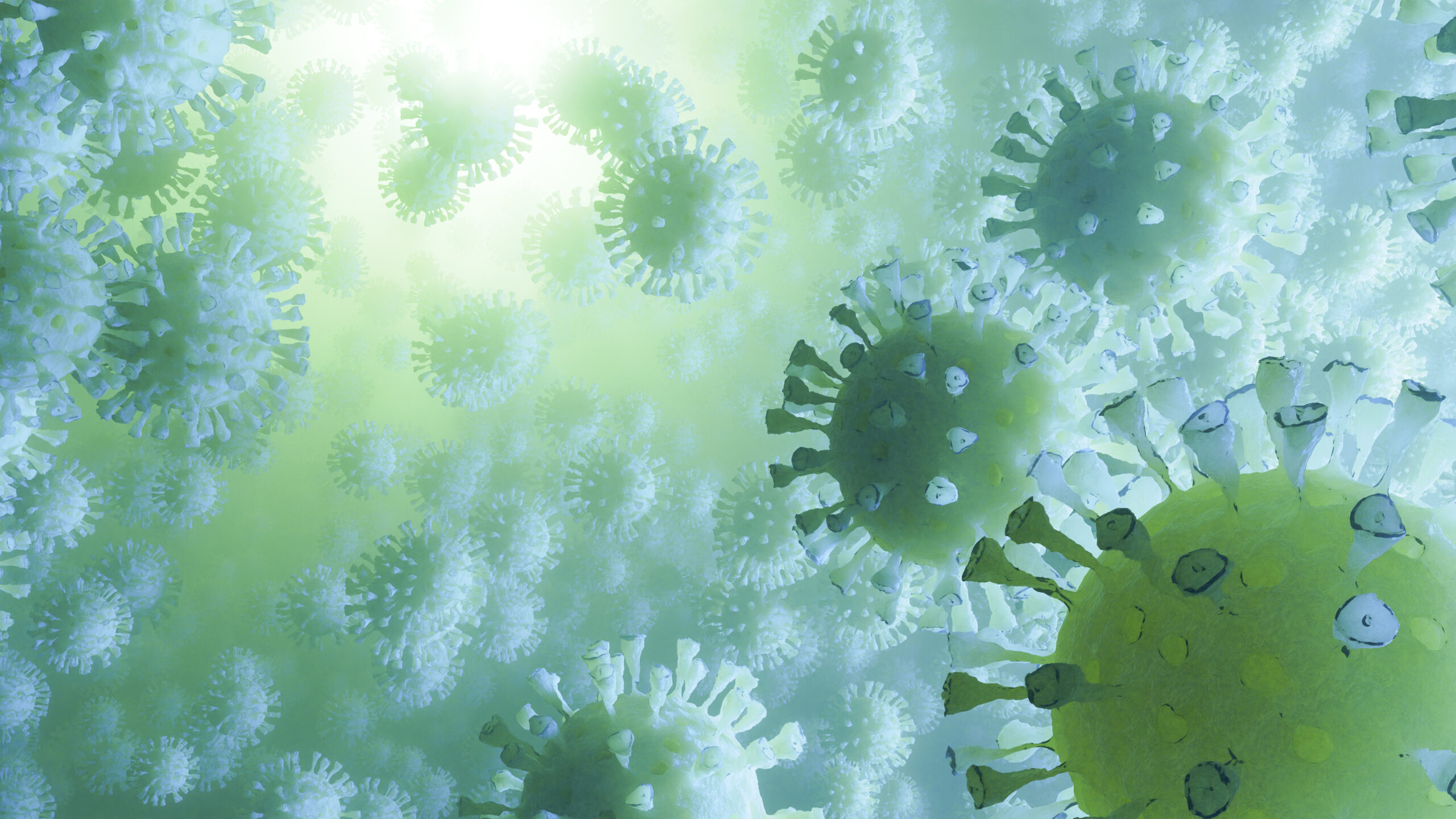
New insights from spatiotemporal analysis
A recent working paper by the Data Science Campus has shown that workers in care homes, warehouses, textile, and meat and fish processing tend to carry the biggest risk of infection from coronavirus (COVID-19) at community level across all urban and rural settlements. This has been a consistent pattern over the whole period of pandemic after controlling for a wide range of socioeconomic and demographic profiles, land use and travel patterns, vaccination rates, and real time mobility. We used a community-level analysis of influences on COVID-19 and a combination of statistical models to provide these insights.
Understanding and monitoring the major influences on COVID-19 infection (number of cases) in communities is essential to inform policy making and evaluate the impact of non-pharmaceutical interventions (NPIs), such as mobility restrictions, closures of some industrial sectors and schools, social distancing and mandatory face coverings in public areas and on public transport. We can also use this analysis to understand potential health inequalities across diverse communities. For instance, it is of policy interest to understand whether the greater risk of infection for warehouse workers is due to the areas where warehouse workers are residing, factors related to the ethnicity of workers in those jobs, or the workplace and type of job.
Data and modelling challenges
Producing a robust analysis of community level influences on COVID-19 incidents presents some major data and modelling challenges. The analysis requires a comprehensive dataset that can cover a wide range of influences, from socioeconomic and demographic profiles, area types, land use features, behavioural responses, and policy interventions, such as those reflected in mobility or vaccination rates.
Methodologically, the analysis should consider the potential interrelations among influences. For instance, the analysis should account for self-selection and spatial sorting where residents choose their residential locations based on their travel attitudes and preferences, or social structure and inequality. As an example, in evaluating the influences on COVID-19 infection risk, an ideal model should distinguish the effect of living in dense urbanised areas from the impact of belonging to a specific ethnicity group that tends to have higher representation in more populated areas.
The analysis should also consider the dynamic nature of the pandemic where influences and impacts changed over time in response to policies, and there were also developments such as the emergence of new variants of virus.
This is a huge challenge, and the requirements are unlikely to be met with any single dataset. Individual and household-level surveys (such as the Office for National Statistics (ONS) Covid Infection Survey) tend to have small sample sizes making it difficult to incorporate a wide range of influences with sufficient temporal and geographical segments. However, they can capture more detailed influences and interactions within communities which makes them a suitable choice for detailed epidemiological analysis and simulation. In addition, community level analysis can better reflect responses to policy interventions, such as changes in mobility patterns in neighbourhoods.
Responding to challenges of performing community-level analysis
In response, we developed a community-level analysis of COVID-19 influences through assembling a large set of static (socioeconomic and demographic profile and land use characteristics) and dynamic (mobility indicators, COVID-19 cases and vaccination uptake in real time) data in England. These data are integrated from a wider range of sources, including telecoms companies (we used anonymised, aggregated O2 Motion data for this study), test and trace data, national travel survey, and Census and Mid-Year estimates at small area geography (LSOA) level.
To tackle the methodological challenges of highly interrelated influences, we have combined different statistical and machine learning techniques, creating a two-stage modelling framework:
- Latent Cluster Analysis (LCA), using the individual-level national travel survey, to classify the country into distinct land use and travel patterns
- multivariate linear regression to evaluate influences at each distinct travel cluster separately
In the stage of selected features for the model, we also adopted Factor Analysis to understand and incorporate the communality across interrelated features. Our model is then split into distinct time periods based on changes in policies or the evolvement in pandemic so that we can evaluate variations over time.
Our findings
Our findings suggest that there exist significant spatial variations in risk influences with some being more consistent and persistent over time.
Specifically, the analysis of industrial sectors shows that communities of workers in care homes and warehouses, and to a lesser extent, the textile and ready meals industries tend to carry a higher risk of infection across all urban and rural settlements and over the whole period of pandemic that we have modelled in this study. This demonstrates the important role of workplaces in defining the COVID-19 risk of infection after accounting for the major characteristics of workers’ residential areas including land use characteristics, vaccination rate and mobility patterns.
We have published a working paper in medRxiv, which contains the full findings from this study. The work has also been recently published in the peer review scientific journal The Royal Society Publishing.