Sharing knowledge for public good at the Royal Statistical Society Conference
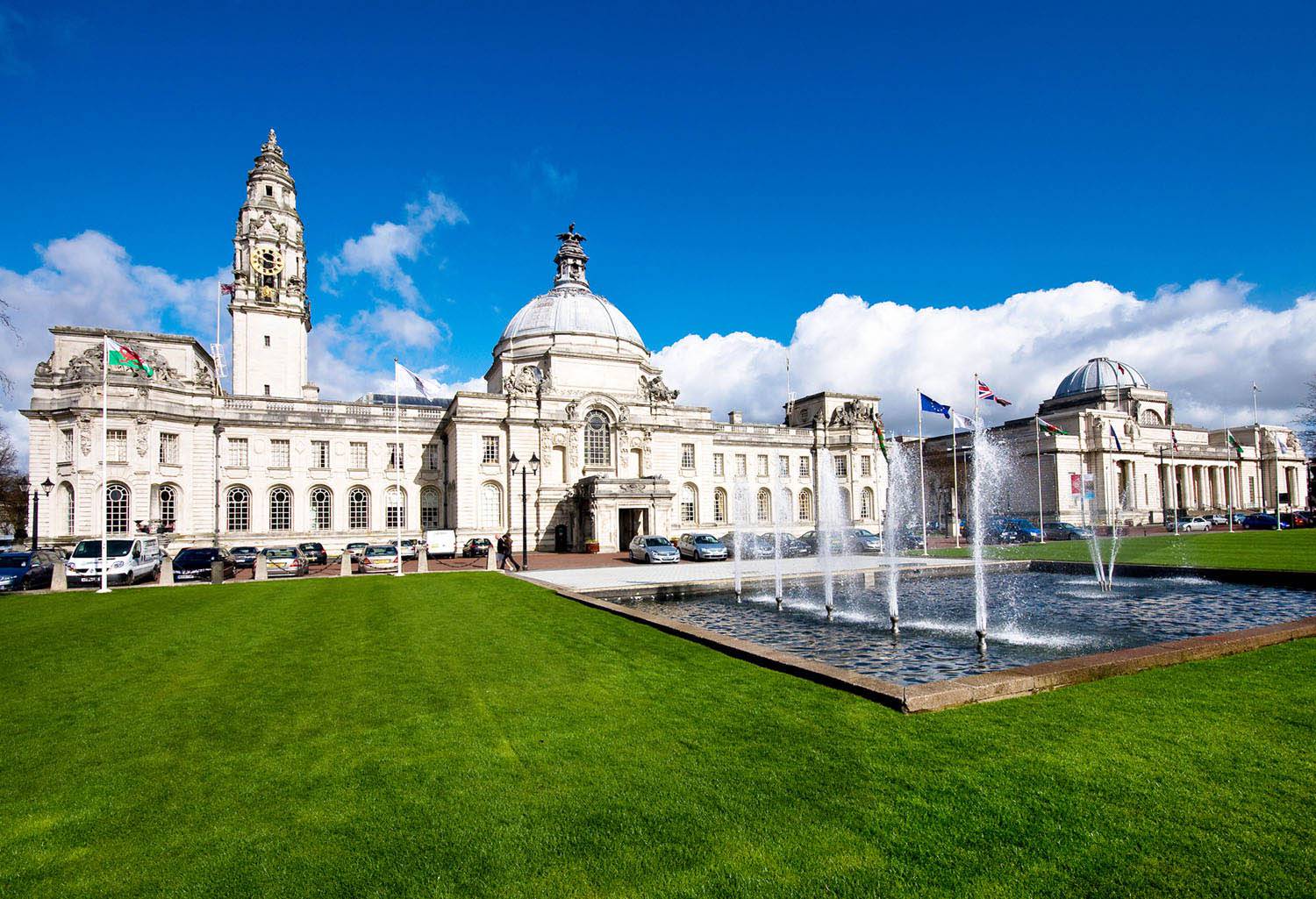
The Royal Statistical Society (RSS) 2018 International Conference was held down the road from our Newport campus in Cardiff’s magnificent City Hall. This annual conference has an increasingly active data science stream sponsored by the RSS Data Science Section, which we at the Campus were keen to be involved with again.
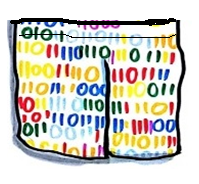
We’d run a traditional invited session in Glasgow at last year’s conference but saw that, in seeking abstracts, innovation in format was welcomed. So, let’s try something a bit different, I thought. We called it “Data Science Shorts”, for no particular reason.
The session we planned provided delegates with the opportunity to explore and comment on current data science issues. We used a knowledge café format: a structured conversational process for sharing knowledge in which groups of people discuss various topics at different tables. Delegates were invited to switch tables periodically, if they wished, and were introduced to the previous discussion at their new table by one of our invited “table hosts”.
We invited table hosts from some of our partner universities and from ONS. On the day, the table hosts were Idris Eckley (Professor of Statistics at the University of Lancaster), Guy Nason (Professor of Statistics at the University of Bristol), Owen Abbott (Head of the Big Data team at ONS), Rowena Bailey (Senior Data Scientist in ONS’s Data Science Campus) and myself. The session was chaired by Tom Smith, Managing Director of the Campus.
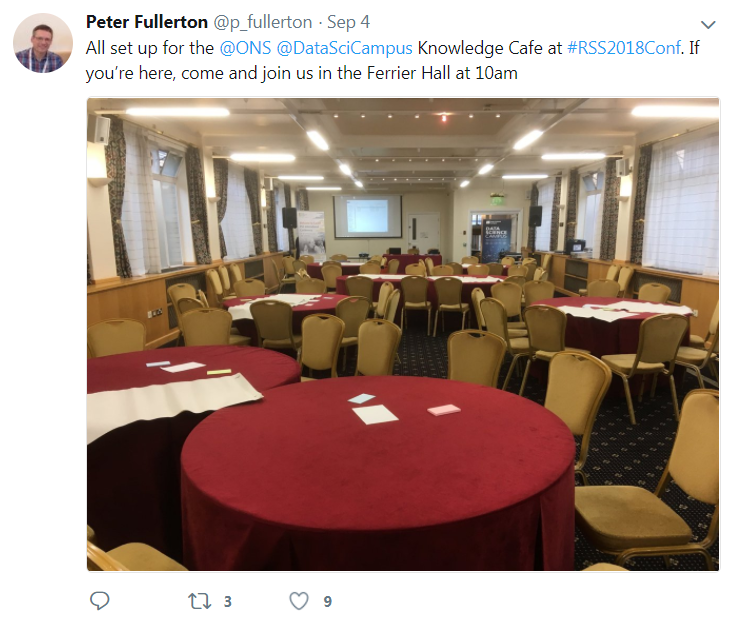
On the first morning of the conference we were all set up in one of the medium-sized rooms, wondering if this was a good idea after all. Would anyone turn up? If they did, would they be willing and active participants? The only pre-determined content was a list of five topics – one for each table:
- How could we enhance the value of collaboration between academia, industry and the public sector in delivering impact from data science research? Host: Idris Eckley
- How could we encourage greater diversity among those attracted to careers in data science? Host: Rowena Bailey
- How can we ensure that data science projects are ethically sound and accepted by the public? Host: Peter Fullerton
- What are the factors underpinning the successful delivery of a data science research project? Host: Owen Abbott
- What are the new horizons for data science – where could there be greatest impact? Host: Guy Nason
I needn’t have worried. For one of the first sessions of the Conference, we had a good turn-out of over 40 people, including the esteemed outgoing RSS Chair David Spiegelhalter. Tom Smith and I described the process, each host introduced the topic to be discussed at their table and off we went. In the 80-minute time slot we had three table “rotations”. Everyone was keen to join in with the discussions and try out different topics. We ended up with copious notes. Each host highlighted some of the main points from the discussions at their table and Tom Smith concluded the session. In haste, I offered to attempt a write-up of proceedings. This is it.
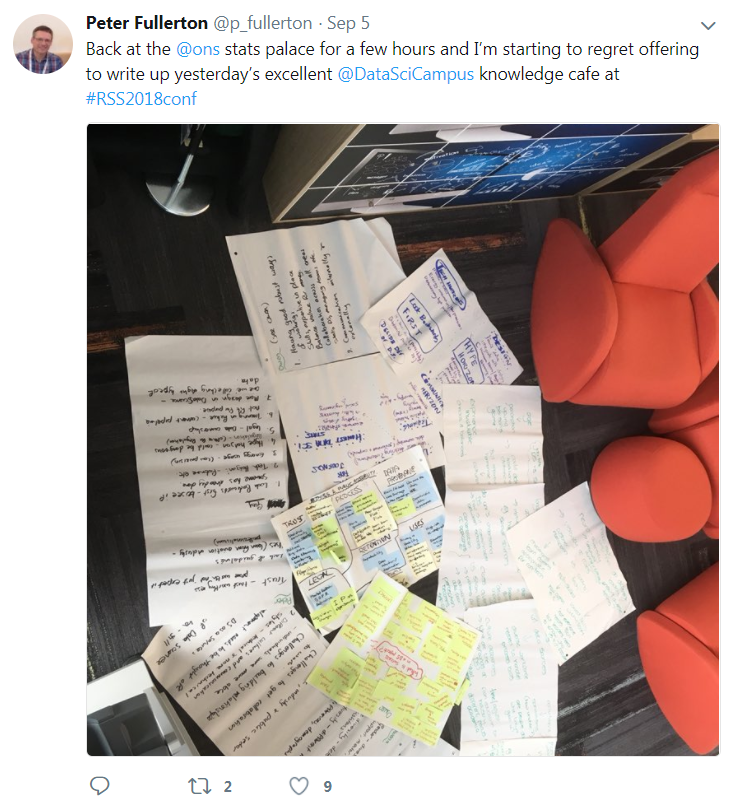
It is difficult to capture the extent and depth of the three discussions at each table. The feedback from participants was positive: they valued the opportunity to hear a range of views from different perspectives, found the format an excellent way of stimulating discussion, and liked the interactive nature of the session. Here is a summary of the main points that arose at each of the five tables.
How could we enhance the value of collaboration between academia, industry and the public sector in delivering impact from data science research?
At this table, the conversations covered partnerships between academia, industry and the public sector and considered the challenges that need addressing to get cross-sector collaborations to be most effective. There are challenges to building relationships – some individuals have a greater aptitude and/or preference for partnership working than others. Different cultures and communication styles – such as technical versus non-technical – could present obstacles too. The discussions identified a need for more development to enhance the value of collaborative working. Intellectual property remains an issue and, despite the legal gateways that exist, many are still wary of sharing data.
How could we encourage greater diversity among those attracted to careers in data science?
The discussion of this topic looked at the relative importance in the recruitment process of communication skills compared with technical skills, recognising the impact of the language used. The diversity of teams’ skills was the goal. The role of leaders in ensuring diverse teams and the value of mentoring and networks were highlighted, as was the importance of culture. Age diversity was a theme raised during the discussion: data science could be perceived as a young person’s game. “Data science” could also deter those with a more statistical background. The discussions at this table also considered neuro diversity – the importance of applying different mindsets to data science, class differences and different demographic groups.
How can we ensure that data science projects are ethically sound and accepted by the public?
The contributions at this table considered the extent of public trust in data science and statistics more widely. There were views that data literacy was lacking and needed to be fostered among users and the public more generally. The need to communicate benefits better was highlighted. The trustworthiness approach championed by Baroness Onora O’Neil, based on intelligent transparency, was referenced: what we do should be accessible, usable and understandable. There is a need to prove that the data science we do is “trustworthy”; we should not just expect public trust. There is a lack of standards and guidelines and no clear approval or other accreditation mechanisms. Could we learn from the professionalism of other industries’ use of algorithms, such as the aviation industry? It is vital to establish a clear provenance for data.
What are the factors underpinning the successful delivery of a data science research project?
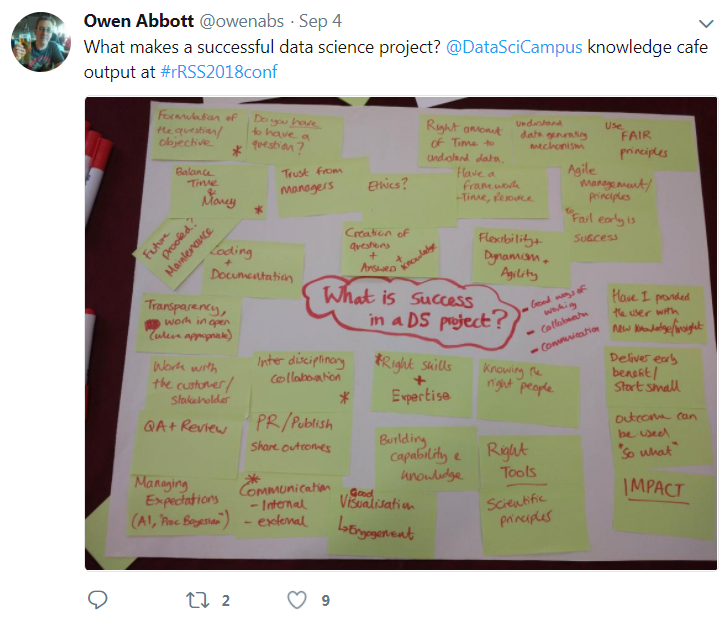
The discussions on this topic explored a broad span of critical factors for delivery. The formulation of the research question or objective from the outset is important, as is allowing sufficient time to understand the data. As with all delivery projects, there needs to be:
- trust from managers
- close engagement with stakeholders and customers throughout the project
- collaboration across all areas
- good, robust ways of working
- effective internal and external communication
Other important factors suggested around the table included the right balance of skills and expertise in place. This should be balanced against value for money, building capability and knowledge, ensuring sufficient quality assurance and peer review and a culture of transparency and openness, backed by appropriate procedures. Innovative data science projects need to be flexible and agile – with a focus on impact, always having the “so what? question in mind. Failing early should be regarded as a successful outcome if there was learning as a result. Where appropriate the outcome should be future-proofed, with maintenance built-in.
What are the new horizons for data science – where could there be greatest impact?
The discussions here looked at a range of different “horizons”, including technical, hype, communications and legal. In thinking about new horizons, it was considered important to look backwards first – to see if someone has already done what you are planning. There are dangers in the hype surrounding data science, linked to a need for the honest and responsible communication of data science and statistical outputs. The participants foresaw a strengthening of legal frameworks and regulation, particularly concerning data ownership and a resultant decline in the free-for-all environment many perceived in the early days of “data science”. An important principle going forward should be a much clearer emphasis on design in data science, relevant data acquisition and the availability of a fit-for-purpose pipeline of training. There was a risk of too much energy being devoted to unnecessary high precision. To paraphrase Einstein, the precision required should be the minimum necessary, but no smaller.
Summary
In summing up the discussion, Tom Smith highlighted some important themes that had emerged across all the tables:
- data science should be a “team sport”, enhanced through collaboration
- the importance of design in data science
- the need for more and better training in data science and an understanding of its value and the risks of using data ethically and responsibly
- there are many lessons to be learned from others in how best to work on a data science project

Overall the event had illustrated the benefit of learning from others, engaging with other sectors, and learning from what has happened in the past
Would I do something like this again? Yes – I was encouraged by how well this format worked in a conference setting. Delegates welcomed the greater opportunity for active participation in discussions. Clearly, we could only scratch the surface of each subject; the topics were often covered in greater depth in the more traditional conference sessions. But I was struck by the great range of experiences from different sectors that we heard about at each table.
Lessons learned? Some more detail up front would allow delegates to think about the topics in advance. And we should really offer coffee in a knowledge café! I’m looking forward to the 2019 conference in Belfast already. We’ll be back.