The use of microdata for firm-level analysis of preference tariff utilisation in the UK: technical report
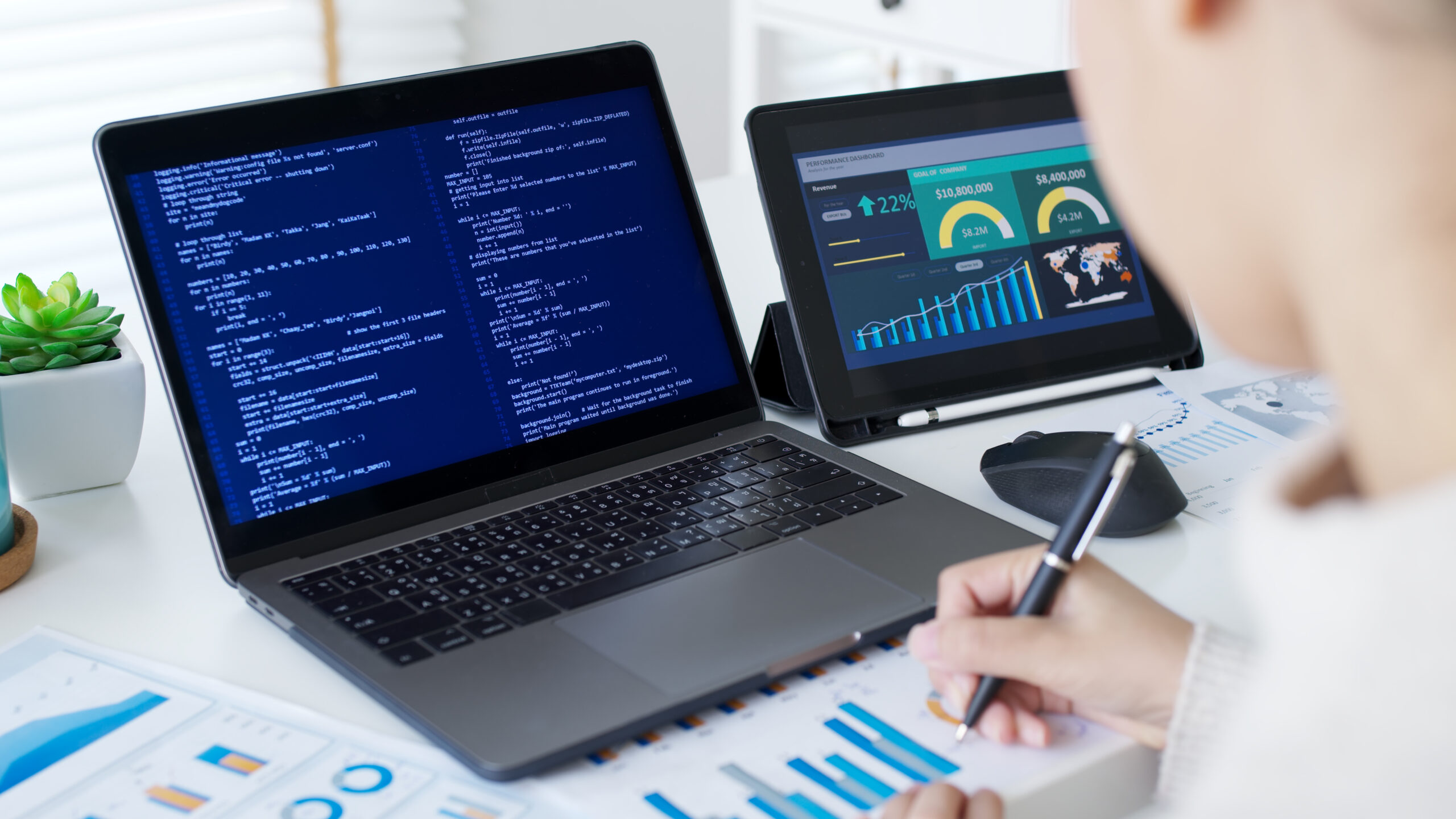
Understanding how trade agreements are used, and how to encourage the use of these agreements, has long been an interest of policymakers. Preferential trade agreements (PTAs) are commonly used by countries to promote international trade. However, a small but growing literature on this topic largely uses aggregate data to analyse PTAs. While existing empirical evidence can provide a general picture on the use of these agreements, it fails to provide knowledge of how the use of PTAs varies across firms and how the pattern of such usage changes over time.
Transactional-level and firm-level data with long time-series coverage are rarely used to analyse preference utilisation rates (PURs). An analysis conducted by the Data Science Campus and the Department for International Trade not only fills a gap in existing literature by using firm-level data from business register and transactional-level customs data to analyse the uptake of preferential tariffs by UK importers from non-EU countries, but it also establishes a longer time series that includes the period from 2009 to 2019.
The HM Revenue and Customs (HMRC) trade in goods (TiG) data made available to us, which include all the relevant information required to conduct our analysis, cover the period ending 2019. HMRC has changed their data collection method since 2021, but our analysis focuses on the period before those changes.
In this technical report, we go behind our analysis on the use of microdata for the examination of preference tariff utilisation and take a deep dive into challenges of drawing together new, administrative data sources to answer policy relevant questions. We also explain the reasons to use firm-level and transactional-level data to analyse PURs and the improvements that our analysis provides as compared with existing empirical studies.
The reasons to use firm-level and transactional-level data for analysis
International trade plays an important role in the UK economy. Total UK trade in goods exports were £366.8 billion in 2019, of which £197.1 billion was from non-EU countries, while total UK trade in goods imports were £541.8 billion in 2019, of which £275.6 billion was from non-EU countries, as described in HMRC’s UK Overseas Trade in Goods Statistics Summary of 2019 Trade in Goods (PDF, 951 KB).
Using data from the GDP quarterly national accounts, UK: July to September 2022 statistical bulletin (PDF, 1825KB) we find that UK exports of goods as a percentage of GDP was 16.24% in 2019, while UK imports of goods as a percentage of GDP was 22.86% in 2019. These figures show the importance of trade as an economic activity, and how increases and decreases in trade activity are likely to have a large impact on the economy.
Wales, Black, Dolby and Awano (2018) report that UK businesses that declare international trade in goods were found 70% more productive on average than those business that do not trade in 2016. It is therefore essential for policymakers to understand what can help to encourage trade activities, how current free trade agreements (FTAs) perform, and how the economic benefits of FTAs could be achieved.
Free trade, or the elimination of trade barriers, can offer economic benefits. These include, for example, more exports, economies of scale, lower prices, and greater choice of products. PTAs enable countries to grant preferential tariffs to imports from their trading partners. Through PTAs, countries give tariffs lower than their most favoured nation (MFN) rates to their trading partners’ products.
These agreements are usually reciprocal in a customs union, which means all parties agree to give each other the benefits of lower tariffs. PTAs can also be offered to a trading partner unilaterally rather than on a reciprocal term. The General System of Preferences (GSP) scheme is an example in which developed countries offer preferential tariffs on imports from developing countries. By analysing the pattern of preference tariff utilisation, policymakers can:
- better understand the effectiveness of trade agreements
- better design policy and operational actions to improve preference utilisation rates (PURs) to ensure UK businesses maximise trade agreement benefits
- learn lessons for developing and implementing new free trade agreements (FTAs) and improve business uptake of preferential trade tariffs accordingly
The full economic benefits of trade agreements can only be achieved if firms make use of preferential tariffs. However, studies show a significant variation in preference tariff utilisation across the member states of the European Union (EU) and other trade partners.
The PUR measures the extent to which preferential tariffs, rather than MFN tariffs, are claimed for preference-eligible dutiable trades. We calculate PURs as the total transaction value of preferential imports divided by the total transaction value of preference-eligible imports.
Using publicly available aggregate data on preference utilisation on EU imports that accompanies a publication on EU trade agreement published by the European Commission, we find that the PUR of imports from all non-EU countries to the UK in 2019 is 82.4%. Although this figure reflects the fact that firms do not always use preferential rates even when they are available, it does not give further information that can help refine the design of policies.
Having a better understanding of how the uptake of preferential tariffs varies across different firm characteristics could help to design policies that target different types of firms. For example, if preference uptakes are found to be quite low among small firms, then policy designed to encourage utilisation among these firms could be beneficial.
Therefore, a detailed breakdown of aggregate PURs is required for better policy, though discovering the reasons behind the uptake of preferential rates is not within the scope of our study. Nevertheless, our findings provide a detailed picture of the uptake of PURs across different types of UK firms, laying the foundation for further investigations.
Information about firm characteristics can either be obtained from surveys or from a business register. Our analysis uses the latter because it has a near-population coverage of UK firms. By linking a business register to transactional-level customs data, we obtain a representative picture of UK traders’ decisions to use preferential tariffs.
As pointed out in National Board of Trade Sweden (2019), details of individual trade transactions are usually hidden in aggregate trade data or trade statistics, whereas transactional-level data provide details of individual transactions or shipments that were declared to customs, the transaction value, frequency, traders’ identifier, and product category.
Combining firm-level information stored in business registers and transactional-level information stored in customs data, as well as a long time-series coverage, our analysis can provide answers to:
- how the use of preferential tariffs changes across firm size over time
- how the use of preferential tariffs changes across product categories over time
- how the use of preferential tariffs changes across regions over time
Our data improvement as compared with existing literature
A combined use of firm-level and transactional-level data to analyse PURs is rare in the growing literature on PURs. Transactional-level administrative data are usually not publicly available and access to them often requires special permission from authorities.
Many existing studies in this topic use aggregate data for analysis. For example, Ando and Urata (2018) use the Trade Compass Database of Deloitte to compute free trade agreement (FTA) utilisation rates as share of imports under FTA schemes in total imports at product level and obtain preference margin as the difference between FTA tariffs and MFN tariffs.
Other studies on the utilisation of trade agreements use data from surveys that are based on defined samples. For example, PricewaterhouseCoopers (PWC) (2018) has conducted a business survey that asks Australian firms about their experience with using FTAs, particularly with China, South Korea, and Japan.
Takahashi and Urata (2008) base their analysis on data from a survey in which 469 firms were asked if they have used FTAs, the reasons for not using them, their experience in the ease of using FTAs, and the impact of FTAs on their sales, costs, and profits.
However, the use of transactional-level data is still quite rare in existing PURs literature. Albert and Nilsson (2016) use transactional-level data to study utilisation of preferential rates. They estimated the fixed-cost thresholds of using preferential tariffs using transactional-level data for EU exports for 2011 obtained from Iceland’s custom authorities.
National Board of Trade Sweden (2019) use detailed-level administrative data, merged with company-level data, to examine the use of the EU’s FTAs in trade transactions with South Korea by Swedish importers in 2016. Their report gives a thorough analysis of how the use of preferential tariffs varies across firm characteristics.
They investigate the importance of tariff preference on total imports; examine PURs across firms, products, and import modes; and obtain transaction values and preference margin by firm size and product. They also use a logit regression to examine the relationship between utilisation of tariff preferences and potential duty saving.
Our study makes two improvements to existing PURs analysis. First, we draw our insights with the aid of data science methods that combine statistical and machine learning techniques. Secondly, we also make improvements in the use of data, which are:
- combining transaction-level custom data with data stored on the UK business register, enabling us to provide a more detailed examination of which firm characteristics play an important role in the firms’ uptake of preferential tariffs
- using administrative data, which allows us to have a large coverage of UK firms that have declared trade transactions to HMRC; as we are not restricted by sample size, we can obtain a more representative picture of preferential tariff utilisation in the UK economy
- covering import transactions for 10 years in our study, allowing us to establish long time series to look at the patterns in PURs over time
In the remaining part of this technical report, we will provide an overview of the microdata available in the UK for our analysis of PURs and the characteristics of these data. We will then discuss the challenges in using these data for our analysis.
Data for firm-level analysis of preferential tariff utilisation in the UK
There are three main datasets that enable firm-level analysis of preferential tariff utilisation in the UK. Two of these, HM Revenue and Customs (HMRC) trade in goods and the Inter-Departmental Business Register, come from administrative records. The third is International Trade Centre’s Market Access Map.
Trade in goods
UK trade in goods (TiG) data are collected by HMRC for administration and tax purposes. Although HMRC has changed their data collection method since 2021, our analysis focuses on the period before those changes. The TiG data cover a large proportion of UK trade in goods transactions and are used by HMRC for two National Statistic series: Overseas Trade Statistics (OTS) and Regional Trade Statistics (RTS). RTS data provide a breakdown of UK imports and exports by UK region and are derived from the OTS. For information about the trade data sources, see this publication by HMRC. The TiG data cover three types of transactions.
Firstly, TiG data cover import and export transactions between UK businesses and those within the European Union (EU); data on UK’s trade in goods with EU member states are collected through VAT return submission of businesses. Businesses with a larger amount of trade, whose monthly value of trade usually crosses an administrative threshold, are required to report using the Intrastat monthly survey; this allows HMRC to collect more detailed data about their trading activity. For more information about the administrative thresholds and the three types of transaction included in the trade data, see also Section 3.3 in Wales, Black, Dolby and Awano (2018).
Secondly, TiG data cover import and export transactions between UK businesses and those outside of the EU; businesses that import and export goods to and from non-EU countries are required to complete a custom declaration, mainly through the Custom Handling of Import and Export (CHIEF) system. These data are regarded as administrative records, unlike those collected from EU imports and exports that are from survey sources.
Thirdly, TiG data cover estimates and adjustments; this includes estimates of total values of trade missing where businesses have not submitted their returns or have submitted incomplete returns, and those accounting for complex VAT fraud. It also includes estimates for businesses that operate below the reporting threshold of Intrastat.
The TiG data contain a rich set of information about each trade transaction that crosses the UK border. Such information includes country of dispatch or destination, value of trade, commodity type as given by the commodity code, trader identifier, custom procedure code, and rate of duty being claimed. Rate of duty being claimed is a major variable in the analysis of preferential tariff utilisation as it indicates whether a preferential tariff is being claimed for the transaction.
The UK TiG data have been used for trade analysis, see for example Wales, Black, Dolby and Awano (2018). They have also been used for statistical publication. But they have not been used for firm-level analysis of preferential tariff utilisation in the UK. Our project is the first in the UK to examine this topic using this transactional-level administrative data.
While the TiG data contain information about trade transactions and information on whether preference tariffs have been claimed, they do not provide information about firm characteristics such as geographical location, turnover, and employment. Using these data alone do not allow us to examine how the uptake of preferential tariffs varies across different firms. There is a need to enrich these data with firm-level characteristics, prompting us to link them to a business register.
The Inter-Departmental Business Register
The Inter-Departmental Business Register (IDBR) was developed in 1995 to replace the Value Added Tax (VAT)-based register of the Central Statistical Office and Pay As You Earn (PAYE)-based register of the employment departments. See Review of the Inter-Departmental Business Register (PDF, 745KB) for more details.
It is a comprehensive administrative record with near-population coverage of UK businesses. The IDBR covers all businesses registered for VAT and/or PAYE schemes, but it does not cover those without VAT (for example, businesses fall below the VAT threshold) or without PAYE schemes (for example, self-employed or without employees). There are about three million live businesses registered on the IDBR as of December 2019.
The register holds business information such as names, addresses, Standard Industrial Classification (SIC), employment, turnover, ownership, and legal status. Such information is drawn from different sources including HMRC VAT and PAYE schemes, Companies House data, Dunn and Bradstreet, and Office for National Statistics (ONS) surveys. A firm presents on the IDBR as different business units.
The first type of business units is called statistical units, which consist of:
- an enterprise group, which is a group of legal units (enterprises) under common ownership
- an enterprise, which is the smallest combination of legal units, generally based on VAT and/or PAYE records, with a certain degree of autonomy in decision-making; it can carry out one or more activities at one or more locations, and it can also be a sole legal unit, in other words, it does not belong to an enterprise group
- a local unit, which is part of an enterprise located in a geographically identified place where all or part of the economic activity of the enterprise is carried out
The second type of business units is called administrative units, which consist of:
- VAT unit; an enterprise can have one or multiple VAT units. In the IDBR, these VAT units are classified into different types, according to the VAT reporting arrangement adopted by an enterprise
- PAYE unit; an enterprise can have one or multiple PAYE units
The third type of business unit is called observation units, which are known as the reporting units. A reporting unit holds the mailing address to which official survey questionnaires are sent. In many cases, a reporting unit and an enterprise are the same in scope — this is true when a business has a simple structure on the IDBR. However, a large enterprise could arrange with the ONS to have separate reporting units.
This is either to alleviate reporting burden or because the enterprise is involved in multiple business activities that belong to different industries according to the Standard Industrial Classification (SIC). For example, a manufacturing enterprise that also involves retail could have a separate reporting unit for its retail division to answer surveys that are specific for retailers.
Figure 1 and 2 display examples of a simple and a complex firm in terms of IDBR units. Different information is stored in the IDBR under each of these units, in the form of data tables. These data tables are known as other government department (OGD) IDBR extracts; they are snapshot data taken from the register at a particular point in time. Those firms with a complex structure as in Figure 2 are usually large firms that are involved in multiple businesses activities. See also Appendix 1 in Lui, Black, Lavendero-Mason and Shaft (2020) (PDF 2367KB) for discussion about the IDBR.
Figure 1: An example of a simple firm on the IDBR
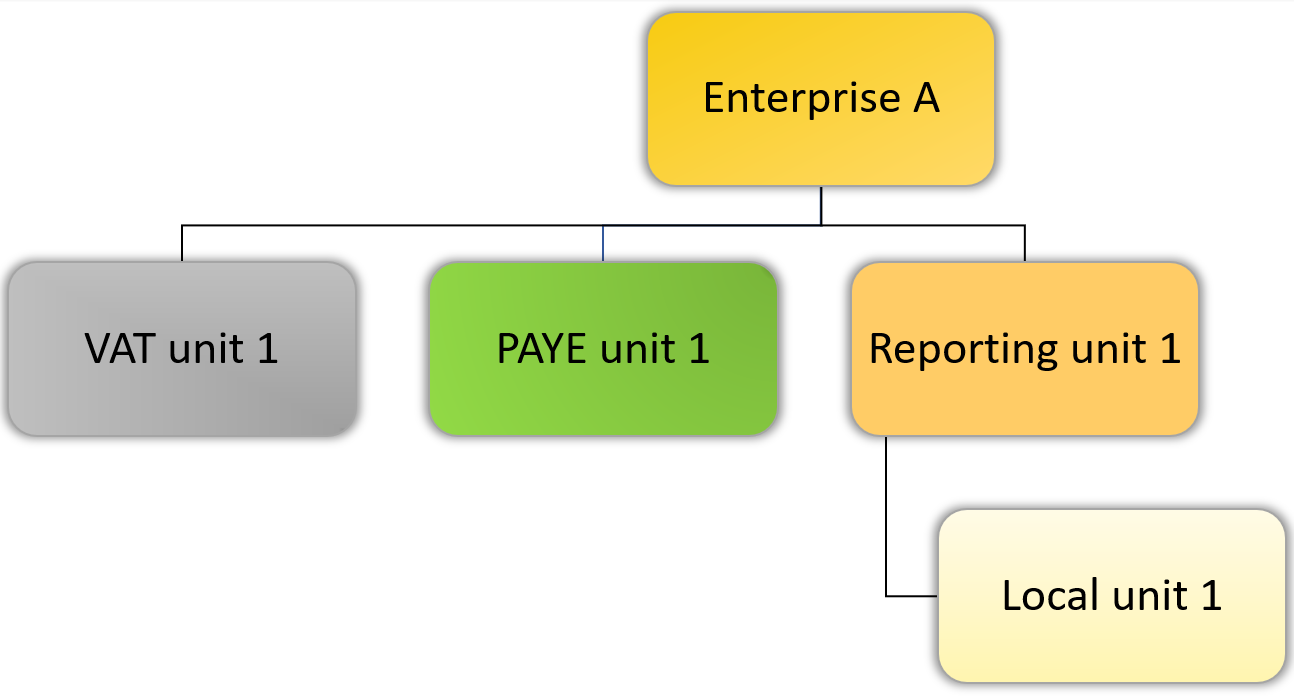
Figure 2: An example of a complex business on the IDBR
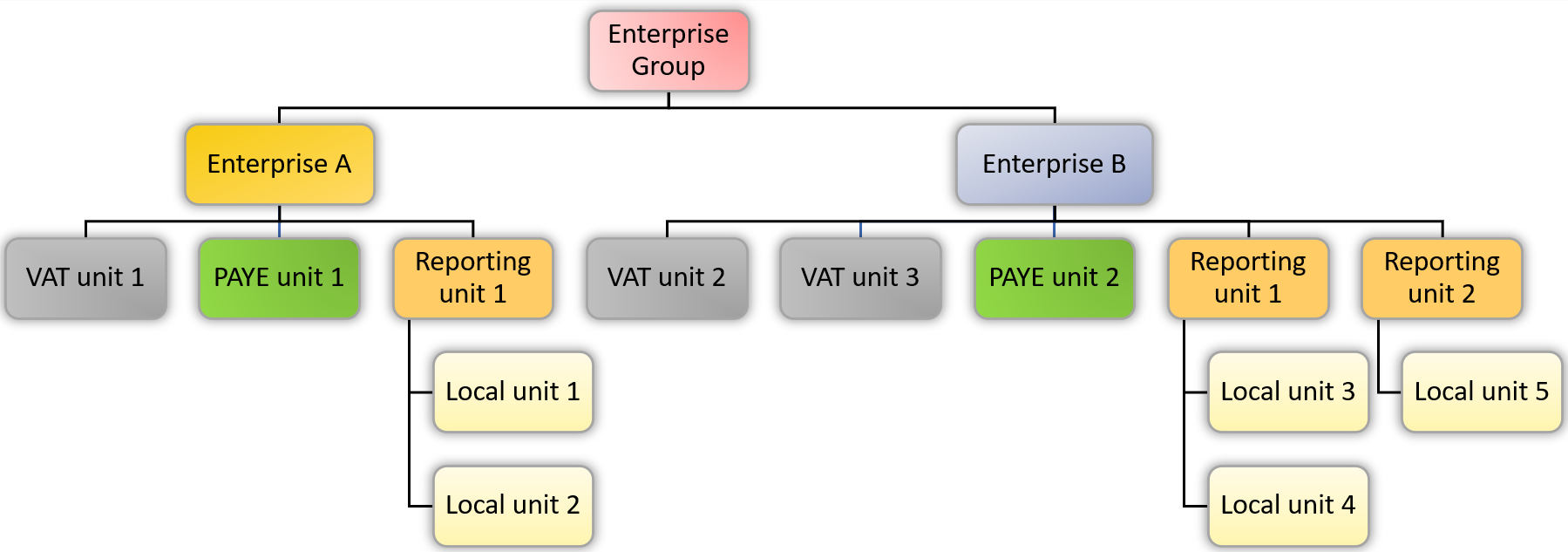
Important information required for firm-level PURs analysis
While TiG data provide details of individual trade transactions, the IDBR contains a rich set of information about a firm, and this information is stored in separate data tables of its IDBR units. For our firm-level analysis, the information stored on the enterprise, reporting unit, and VAT units suffice. Figure 3 summarises the important information required from the two datasets for our study.
Figure 3: Important information in the TiG and IDBR datasets for firm-level PURs analysis
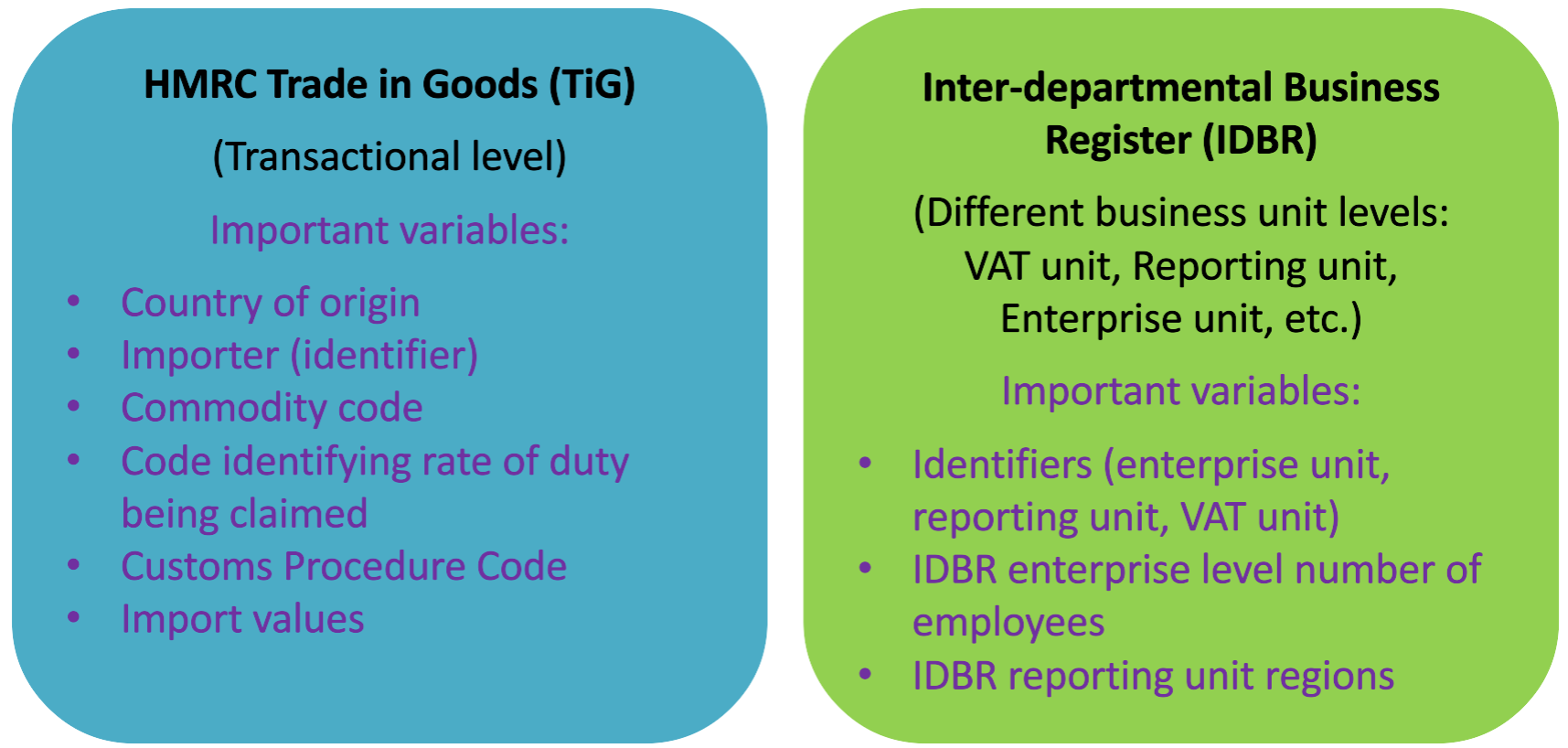
The TiG data provide us with information about trade transactions declared by the VAT trader. The IDBR provides us with information about businesses. Our project makes use of the data about firm characteristics stored in the IDBR enterprise, reporting unit and VAT unit tables. We produce a linked IDBR dataset to gather details about firms stored in its separate business units using different IDBR identifiers. The TiG dataset can be merged with the linked IDBR dataset using the VAT reference number as the common identifier.
Construction of the IDBR-TiG linked dataset
Our firm-level analysis focuses on the uptake of preference tariffs of UK imports from non-EU countries. We make use of the IDBR and TiG administrative datasets. A linked IDBR-TIG dataset is constructed to investigate how different firm characteristics relate to businesses’ uptake of preference tariffs on import transactions.
Figure 4 summarises the construction of the IDBR-TiG linked dataset, we start the procedure by first combining the IDBR enterprise, reporting unit and VAT unit data tables using the enterprise reference number as a common identifier to create a linked IDBR dataset. We then merge the TiG data to this linked dataset via VAT reference number.
Figure 4: Construction of the IDBR-TiG linked dataset
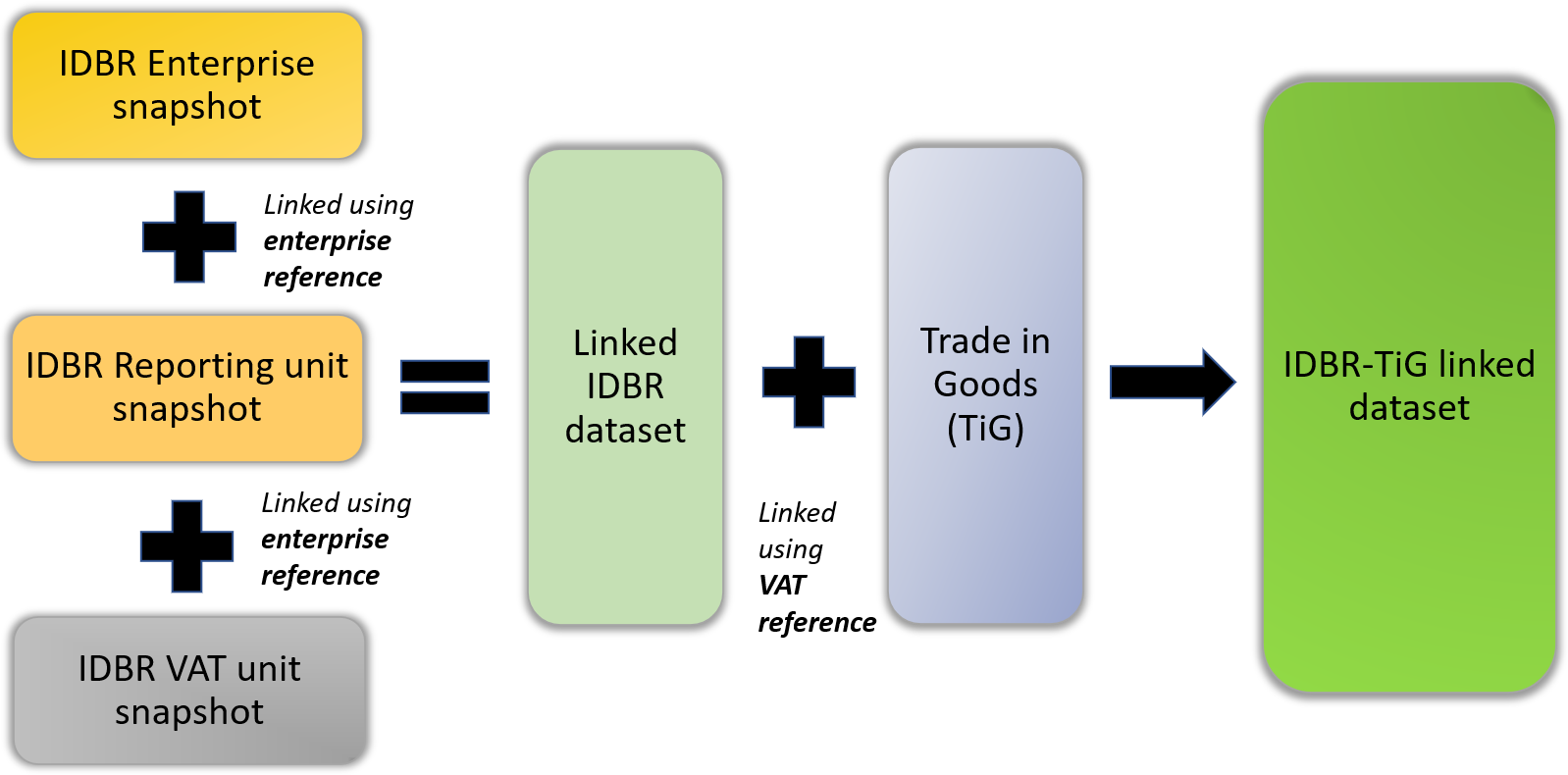
Combining these two datasets would enable us to conduct detailed-level analysis. However, the linking process is not a straightforward exercise because of some data limitations. To establish an appropriate strategy to combine these data, it is crucial to understand these limitations. Moreover, a good knowledge of these issues and the challenges involved in using these data for firm-level analysis also enable us to understand the caveats to our findings. In the next section, we discuss the challenges we face in using these data.
Market Access Map
We need to identify the import transactions from the IDBR-TIG linked data that are eligible for preferential tariffs, as these are required for the estimation of preference utilisation rates, based on the definition we gave earlier. We achieve this by using the International Trade Centre’s Market Access Map (MAcMap), which is a database that provides information such as customs tariffs, tariff rate quotas, regulatory requirements and preferential tariffs that apply, for each product.
We linked the IDBR-TIG database to MAcMap by matching the commodity code from IDBR-TIG to the product code from MAcMap at the 10-digit commodity level. Then, we removed all transactions not eligible for preferential tariffs by dropping transactions that do not have a corresponding preferential tariff rate in MAcMap based on the year, country and commodity code.
The challenges in using UK microdata to analyse PURs
The TiG data and the IDBR are commonly known as administrative data or administrative records. Administrative data are not designed for scientific research but are records created during some administrative operations. They can be used for statistical production, for example, the Overseas Trade in Goods Statistics published by HMRC.
The compilation of statistics based on administrative sources is increasingly common. For example, the HMRC VAT returns data have been used for the production of national accounts, including short-term indicators and for gross domestic product (GDP).
Administrative records are also used for other statistical operations, for example, the IDBR is the sampling frame of ONS business surveys. However, it is not until recent years that the use of administrative data for scientific research has become popular.
There are pros and cons of using administrative data for analysis, and there are also challenges involved (see Hand (2018) and Künn (2015) for a discussion of some of those issues). Access to administrative data usually requires special permission granted by official bodies. These data are not restricted by sample size and usually have population or near-population coverage.
Hence, they allow us to build a representative picture of an economy or of the entities in the economy (for example, businesses and individuals). However, administrative data are also subject to human errors or misreporting. Quite often, these errors are not easily identified, and it is very difficult to verify the accuracy of administrative data. The Rotterdam Effect (which we are about to discuss) is an example of misreporting that affects official statistics.
We focus our discussion here on the challenges specific to our study of preferential tariff utilisation in the UK using the data sources discussed in the previous section.
Challenge 1: The Rotterdam effect (or the transhipment effect)
The Rotterdam effect (also known as Rotterdam-Antwerp effect or transhipment effect) refers to the problem of misreporting trade when goods pass through major ports on their way to their final destinations. This problem distorts calculation of trade statistics.
Rotterdam and Antwerp are import hubs for trade from non-EU countries before it is routed to other EU destinations. Misreporting occurs when, for example, goods trade flowing from Africa to the UK stops at the Netherlands for a short period of time and is wrongly recorded as UK trade with the Netherlands.
Existing research has found a significant quantity of Dutch imports from EU and non-EU countries that were re-exported to the UK (see Lemmers (2019)). Moreover, using HMRC data from 2013, the ONS has estimated the impact of the Rotterdam effect on UK trade estimates with EU and non-EU countries compared with the actual published data; this showed that the Rotterdam effect might mean exports to non-EU countries are higher by 4.3 percentage points (from 49.6% to 53.9% of total trade in goods) and imports from non-EU countries higher by 4.2 percentage points (from 46.7% to 50.9% of total trade in goods).
These estimates are based on an extreme assumption that 50% of UK trade with the Netherlands is related to non-EU countries; see Table 2 of the UK Trade in Goods estimates and the ‘Rotterdam Effect’ publication.
The Rotterdam effect potentially affects the representativeness of any analysis using UK trade data, whether the focus is on imports from non-EU countries (underestimation) or imports from the EU (overestimation). Our study examines the uptake of preferential tariffs in UK imports from non-EU trading partners for the period 2009 to 2019. The Rotterdam effect implies that the TiG data on UK imports from non-EU trading partners may not include all UK import transactions with the non-EU countries.
Developing methods to produce better estimates in the light of the Rotterdam effect is beyond the scope of our study. There are existing studies on developing methods to produce better estimates of the share of imports that are destined for re-export; see, for example, Lemmers and Wong (2019). Further analysis of PURs could make use of these methods.
Challenge 2: Gathering relevant information for firm-level analysis is a challenging task
Firm-level analysis requires information stored in the different IDBR tables, and each of them is specific to a business unit of the same firm (enterprise, reporting unit, and VAT unit). Figure 1 and 2 illustrate how these different units of the same business relate.
It is important to note that not all identity links displayed in the two figures are directly observed. For example, there is no direct link between the VAT unit, through which the TiG data are linked to the IDBR to create a dataset for our analysis, and the reporting unit that contains certain firm-level information.
This is because, according to the IDBR structure, administrative units are directly related to or sit underneath the parent enterprise of their administrative headquarters, but they are not the part of the firm that is responsible for answering surveys.
Moreover, different information is stored in different IDBR tables that are specific to the business units. That is, not all firm-level information of interest to our analysis is stored in the data tables of a single IDBR business unit. This is mainly because of how information is stored on the IDBR and from which channel the information is collected.
For example, details about location and employment are not stored in the IDBR VAT data tables. While the IDBR reporting unit data tables contain reporting unit-level geographical information, this is not available in the enterprise data tables. Besides, information on the IDBR is not real-time or near real-time, even though administrative information is usually updated on an as-and-when basis. There is often a time lag in the arrival of information.
Pooling information recorded in different business units within a firm is a challenging exercise. It requires us to first construct an IDBR-linked dataset to combine the enterprise, reporting unit, and VAT unit data tables to re-establish the “lost” identity links within the same firms. Once this is done, we can then match the TiG data to the linked IDBR dataset.
Pooling data stored in different IDBR business units also requires us to understand the limitations of the information derived from these different business units. For example, whether this information applies to the whole firm or only part of the firm. This concern arises as not all firms on the register have the simple structures shown in Figure 1. Many firms on the register have complex structures similar to Figure 2, with multiple business units at levels below the enterprise. We will explain in more detail the complications this causes.
Challenge 3: The definition of a “firm” can vary
The concept of “firm” appears to be quite elastic among existing firm-level analysis using the IDBR. For example, Lui, Black, Lavendero-Mason and Shaft (2020) (PDF 2367KB) study business dynamism at the enterprise level, while the ONS regional productivity analysis uses the Annual Business Survey local unit dataset. Hence, a “firm” in an existing study could refer to a local unit, a reporting unit, an enterprise, or a VAT unit. It depends on which IDBR unit(s) the study draws its data from.
This is not an issue if the analysis only requires information related to one IDBR business unit, and if an analysis focuses on firms with simple structures (as depicted in Figure 1). In this case, an enterprise has only one reporting unit, one local unit, one VAT unit, and one PAYE unit. Therefore, information stored in any of these data tables is applicable to the whole firm. However, many firms have complex structures like the one in Figure 2.
In such cases, values directly related to one of the firm’s reporting units or one of its VAT units are not applicable to the entire firm. An import transaction in the TiG data refers to a VAT trader rather than the whole firm, but firm characteristics derived from the IDBR do not always refer to a specific VAT trader.
Our study adopts the assumption that an enterprise (headquarter) is the unit that makes the decision to trade. Therefore, when measuring firm-level characteristics, we prefer information, such as employment, region, and turnover, that is measured at the enterprise level. However, as previously mentioned this is not always possible.
While the IDBR enterprise data table stores the enterprise-level number of employees primarily obtained from the Business Register and Employment Survey (BRES), region is only available at the reporting-unit level. Therefore, for enterprises with multiple reporting units with different region values attached to them, our UK regional results are indicative.
Note that the IDBR also contains enterprise groups each consisting of multiple enterprises. However, not all enterprises belong to an enterprise group. Our study defines an enterprise as the highest level IDBR business unit.
Turnover values are currently stored in the IDBR enterprise, reporting unit, and VAT tables. The source of such values in the enterprise and reporting unit data table are mainly from the Annual Business Survey (ABS) and HMRC VAT source if the firms are not sampled. The ABS contains about 62,000 firms each year. The survey covers all large firms with more than 250 employees with a random sample of smaller firms (including small- and medium-sized firms).
The source of the turnover values stored in the IDBR VAT data tables are mainly from the HMRC VAT returns. Data from HMRC VAT returns are usually a preferred option over data from surveys as the former are more up to date and provide a consistent source of data for all firms. However, as well as having complex business structures, some firms also have complex VAT reporting structures. A complex VAT reporting structure is commonly observed for those enterprises that participate in multiple business activities. There are usually four different types of VAT reporting arrangements.
Type 1: An enterprise has one or multiple standard VAT units. Each of these units reports turnover for the enterprise.
Type 2: An enterprise is a member of a VAT group; that is, multiple enterprises share common VAT registration. A VAT group contains a representative VAT unit and some non-representative VAT units. Only the representative VAT unit reports for all the enterprises in the group. Representative VAT units report turnover to HMRC for the whole VAT group, but non-representative VAT units do not report to HMRC. The proportion of turnover reported by the representative VAT unit for each enterprise is unknown as these shares are not required in the submission to HMRC.
Type 3: An enterprise has divisional VAT units. These VAT units could be found in a large firm that carries out its businesses through a number of divisions. These VAT units share the same Companies House number and hence should not be treated as individual VAT traders.
Type 4: An enterprise has standard VAT unit(s) and, at the same time, belongs to a VAT group.
For the first and third arrangement type, aggregation of VAT turnover to the enterprise level would be straightforward. However, obtaining enterprise-level turnover arrangements for the second and fourth type requires an accurate apportionment strategy. Establishment of such a strategy is beyond the scope of our analysis. Therefore, we take the decision to use turnover values from the IDBR enterprise data table, fully aware that these values are from a mix of survey and administrative sources.
Besides, there is also another complication because of inconsistent VAT reference numbers in the two datasets. The VAT reference number in the IDBR has 12 digits with the last 3 digits constituting the sub VAT number (SVN) that is used to distinguish the representative VAT unit from the non-representative ones. However, VAT traders in the TiG data are only identified by 9-digit VAT reference numbers. This requires us to convert the 12-digit VAT reference in the IDBR data tables prior to merging the register with the TiG data.
Conclusion
The firm-level analysis of preferential tariff utilisation conducted by the Data Science Campus and the Department for International Trade is the first of its kind in the UK that makes use of a rich set of information derived from administrative records. We explain in this technical report what drives us to study PURs using firm-level and transactional-level data, as well as the research questions these data allow us to answer that cannot be answered using aggregate data or statistics.
We provide a brief overview of the data used in existing empirical studies that are largely aggregate data and survey-based data. We then explain the data improvements we have made in our study, along with some of the challenges we have encountered in making those improvements.
We discuss the two UK administrative datasets, the trade in goods (TiG) and the Inter-Departmental Business Register (IDBR), used in our study. Our analysis focuses on UK import transactions form non-EU trading partners. We first construct an IDBR-linked dataset to bring together firm characteristics recorded in the data tables of each unit.
The variables we take from the IDBR indicate employment size, geographic location, and turnover. We then link the IDBR to TiG data and use certain variables in the TiG data that give us details about whether a transaction has claimed preferential tariffs, its importer as identified by Value Added Tax (VAT) reference number, its value, the custom procedure involved, and its country of origin (or dispatch).
There are limitations of the two sets of administrative records that we use. These issues include, for example, misreporting in trade data, “lost” identity links among IDBR business units, updating issues of the IDBR, and complications arising because of complex business structures and complex VAT reporting arrangements. Some of these issues we attempt to address in our study. For example, constructing a linking strategy to re-establish identity links among business units and a strategy to link TiG to IDBR in the face of an inconsistent format of VAT identifiers in the two datasets.
We recognise that some improvements to the data could perhaps be made in future analytical work to increase the quality of microdata for this kind of analysis. For example, future work could establish an appropriate turnover apportionment strategy to better deal with the issues we face with enterprises with VAT group reporting arrangements and to use HM Revenue and Customs (HMRC) VAT turnover data.
We also recognise that some of the data issues are because of how the administrative data are collected and recorded, and hence cannot easily be resolved by researchers and analysts. However, as administrative data are now routinely used in analysis and policymaking, those with responsibility for collecting administrative data should consider the wider public benefits that changes in collection and database design might yield.
Regardless of the data challenges we faced, our preference utilisation rates (PURs) analysis shows how detailed-level administrative data can be used to conduct firm-level analysis of preferential tariffs and how data science techniques can be successfully applied to analyse these questions — laying the foundations for further analysis of preference utilisation in the UK.