Synthetic data for public good and art
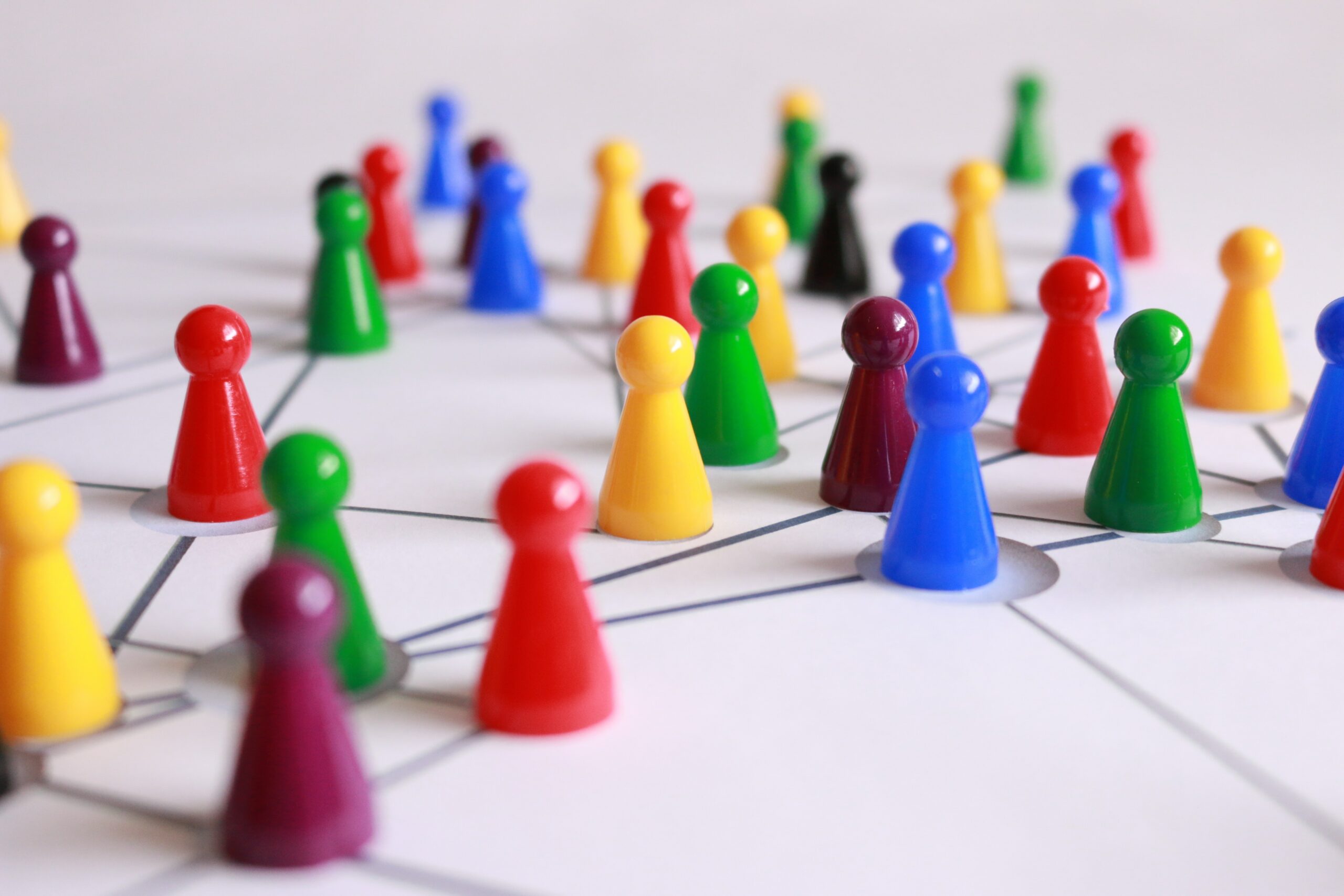
There is an increasing interest in the production of synthetic data across the public sector and beyond.
Government organisations, businesses, academia, members of the public and other decision-making bodies require access to a wide variety of administrative and survey data to make informed and accurate decisions. However, the collecting bodies are often unable to share rich microdata without risking breaking legal and ethical confidentiality and consent requirements.
This can often hinder the efforts of the data science community in providing more detailed and timely analysis to decision-makers to effectively tackle major challenges such as climate change, economic deprivation and global health.
To address this, we have developed a methodology that generates synthetic data – data manufactured artificially rather than obtained by direct measurement.
Synthetic data mimics essential characteristics from the original dataset, but creates new, substitute data that does not represent any real person, removing confidentiality requirements. This makes it suitable for processing and analysis, but without compromising legal, ethical and confidentiality requirements that would prevent the real data from being shared.
Today we present the findings of our work, a complete system for synthetic data generation:
Synthetic data for public good
In our main report, we provide an overview of our initial analysis and propose a system that generates synthetic data to replace real data for the purposes of processing and analysis.
Generative adversarial networks (GANs) for synthetic dataset generation with binary classes
A complementary technical report with a more in-depth coverage of generative adversarial networks (GANs), one of the methods tested for synthetic data generation.
GANs are more than a mathematical curiosity. Recently, the techniques were used to create the 2018 painting “Edmond de Belamy”, which was sold for $432,500! This opened up a potentially lucrative avenue for the wider artificial intelligence (AI) industry.
In the future we plan to develop this work to include datasets with more complex variables, incorporate privacy preserving mechanisms and apply the methods tested here to large-scale datasets. If successful, the methods could be applied to sensitive datasets, to produce non-confidential data that can be used to provide more timely analysis to decision makers in important policy areas.
For more information about this work, please contact the Data Science Campus.
2 comments on “Synthetic data for public good and art”
Comments are closed.
Hi,
I am interested in knowing more of synthetic data generation. May I have more information about this work?
Thank you very much!
Best,
Shumin
Good morning Shumin. Glad to hear you are interested in the work. Can you email us at datasciencecampus@ons.gov.uk with more detail of what you’d like to know and we can put you in touch with those who worked on the project? Thanks