Using open-source data to measure our engagement with the natural environment
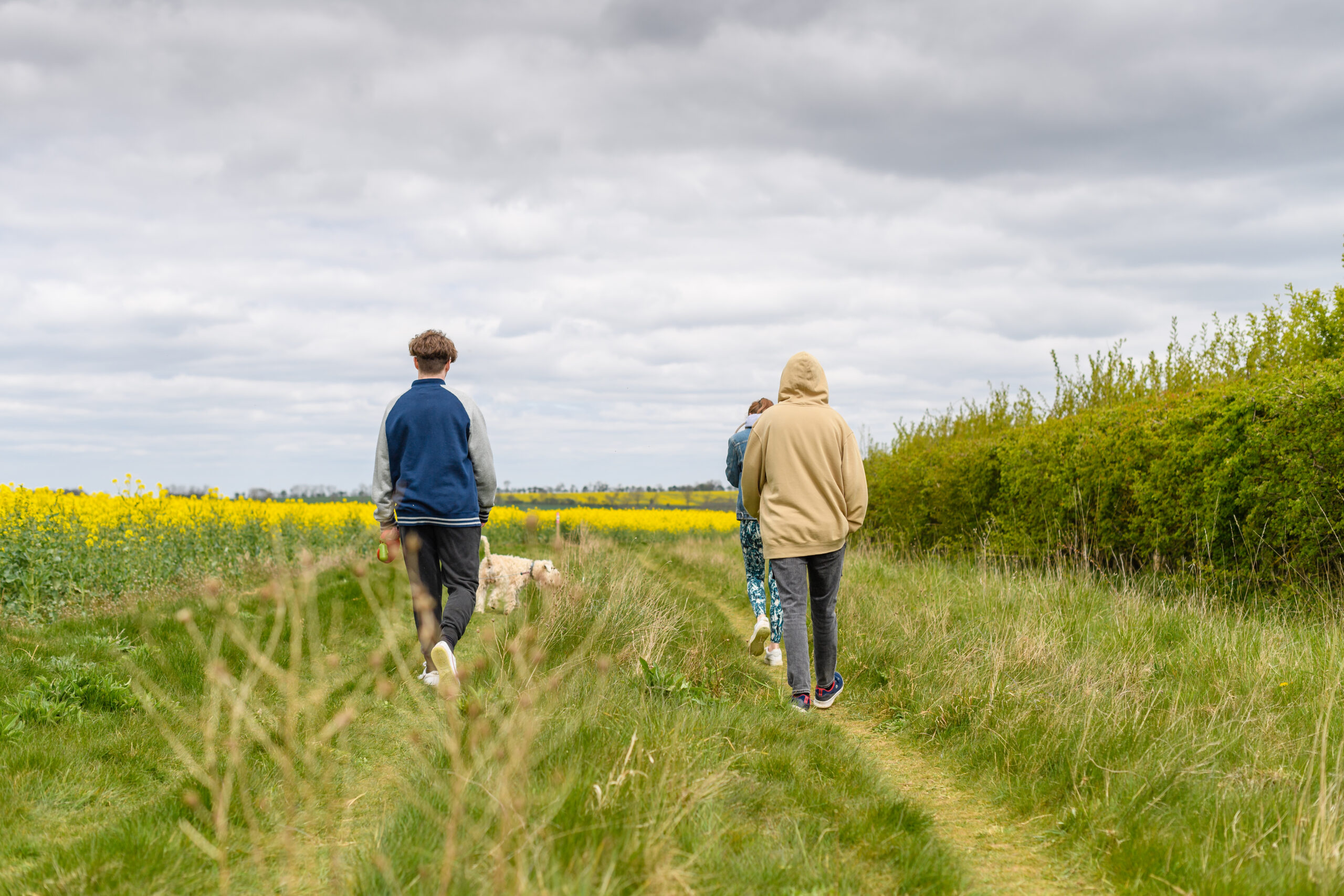
Using freely and openly available data, ONS Data Science Campus (DSC) and Defra’s Spatial Data Science team developed a novel solution for estimating the number of visitors to natural spaces across England. This evidence supports understanding of progress against Defra’s Environmental Improvement Plan 2023 which sets out key targets and commitments on access and engagement with nature, including a commitment that everyone should live within 15 minutes’ walk of a green or blue space. The initial results are promising showing that a reasonable performance can be achieved.
To track progress on nature engagement, Defra has a set of indicators. The current indicators use data from Natural England’s People and Nature Survey which asks a nationally representative sample of 25,000 adults how often they spend time in the natural environment. Due to limitations in sample size, the data from this survey is not useful on small areas.
The number of outdoor recreation and tourism visits are also made available for the UK and the four nations as part of ONS’ UK Natural Capital Accounts. These estimates are also based on survey data and are broken down by habitat types, but do not provide estimates for specific locations.
Automated people counters are used frequently to monitor pedestrian and cycling activity with a good temporal resolution. However, people counters are expensive to install and maintain and for this reason are only installed in a few strategic locations.
Introducing an inexpensive and widely applicable data science method for monitoring visitor numbers would considerably enhance Defra’s indicator for tracking nature engagement. DSC, Defra and Natural England have spent the last few months developing a novel, low-cost data science solution to measuring the number of people who visit England’s natural spaces.
Our model combines aggregated and anonymised data from Strava Metro with carefully selected open or free-to-access spatial datasets such as automated people counters and indicators of local environmental and social conditions. These results are experimental, in order to produce more robust results to inform outcomes we need to incorporate a larger set of training data and datasets that cover the residential location of visitors.
Process
Figure 1 shows the process involves fusing a set of different datasets to develop a data science model, calibrating the model, evaluating the quality of estimation, and testing the model. This is an iterative process requires revisiting assumptions, incorporating new datasets, or selecting different techniques to improve estimation and testing the outcomes.
Figure 1: Different steps involved in the development of a data science approach to estimate Monthly Average People Count (MAPC) in diverse natural spatial locations.
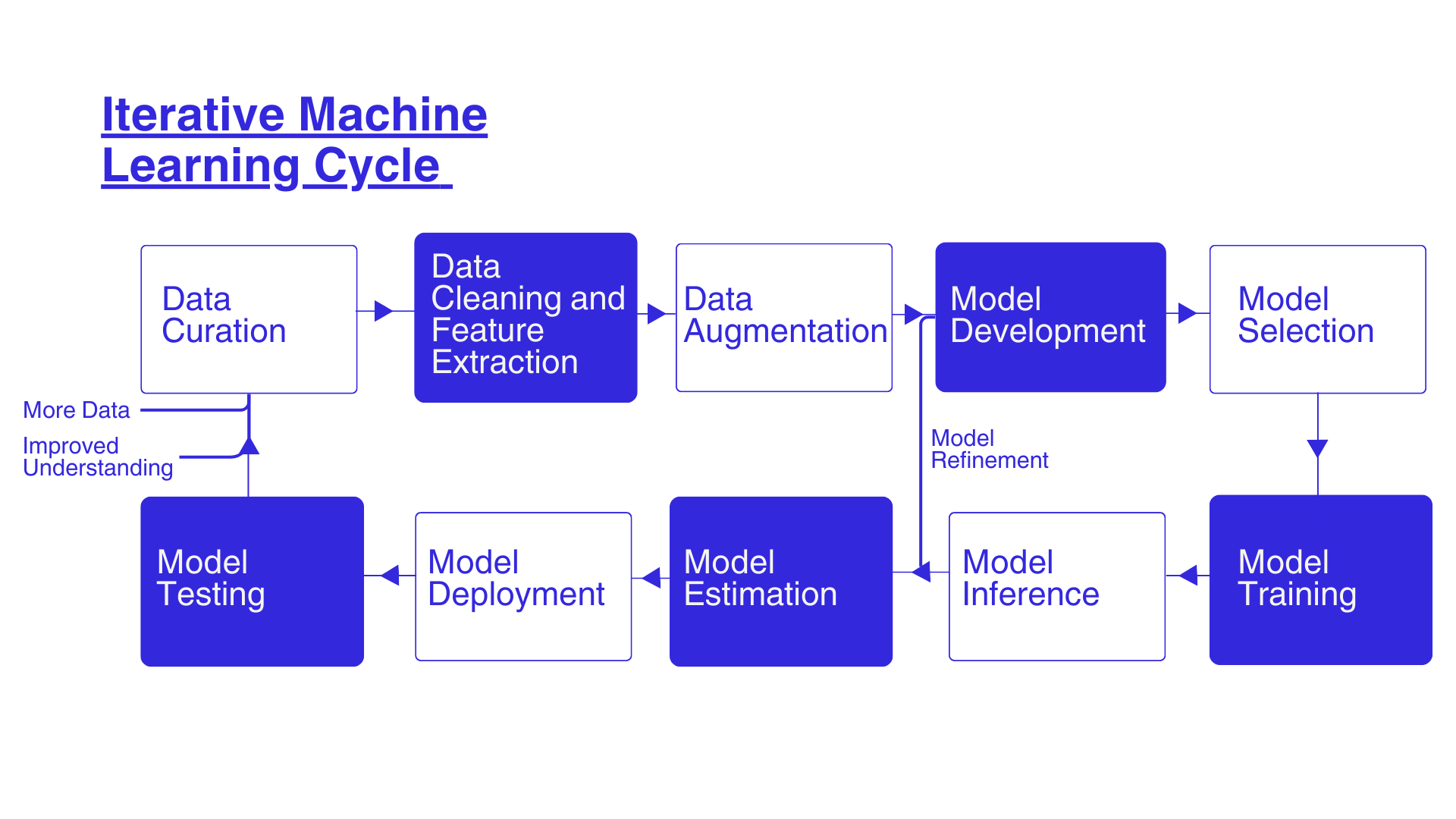
Data Sources
We have provided a brief explanation of the data we used for developing the model. Automated people counter data is used as the target variable against which we evaluated our model performance. The other datasets are used as input to the model for estimating monthly average people count.
Automated people counter data:
Natural England’s National Trails and the North Downs Way together have a good network of 36 automated people counters to monitor trail usage. The data from these counters was used as the ground-truth data in our model. As the quality of data from these people counters is variable only data from selected sites that met out data quality threshold was included. The approximate locations of people counters are shown in Figure 2.
Figure 2: People counter locations and respective data providers. The exact location of all people counters in the map have been obscured by moving the original location by a random distance between 5km and 10km and by a random angle.
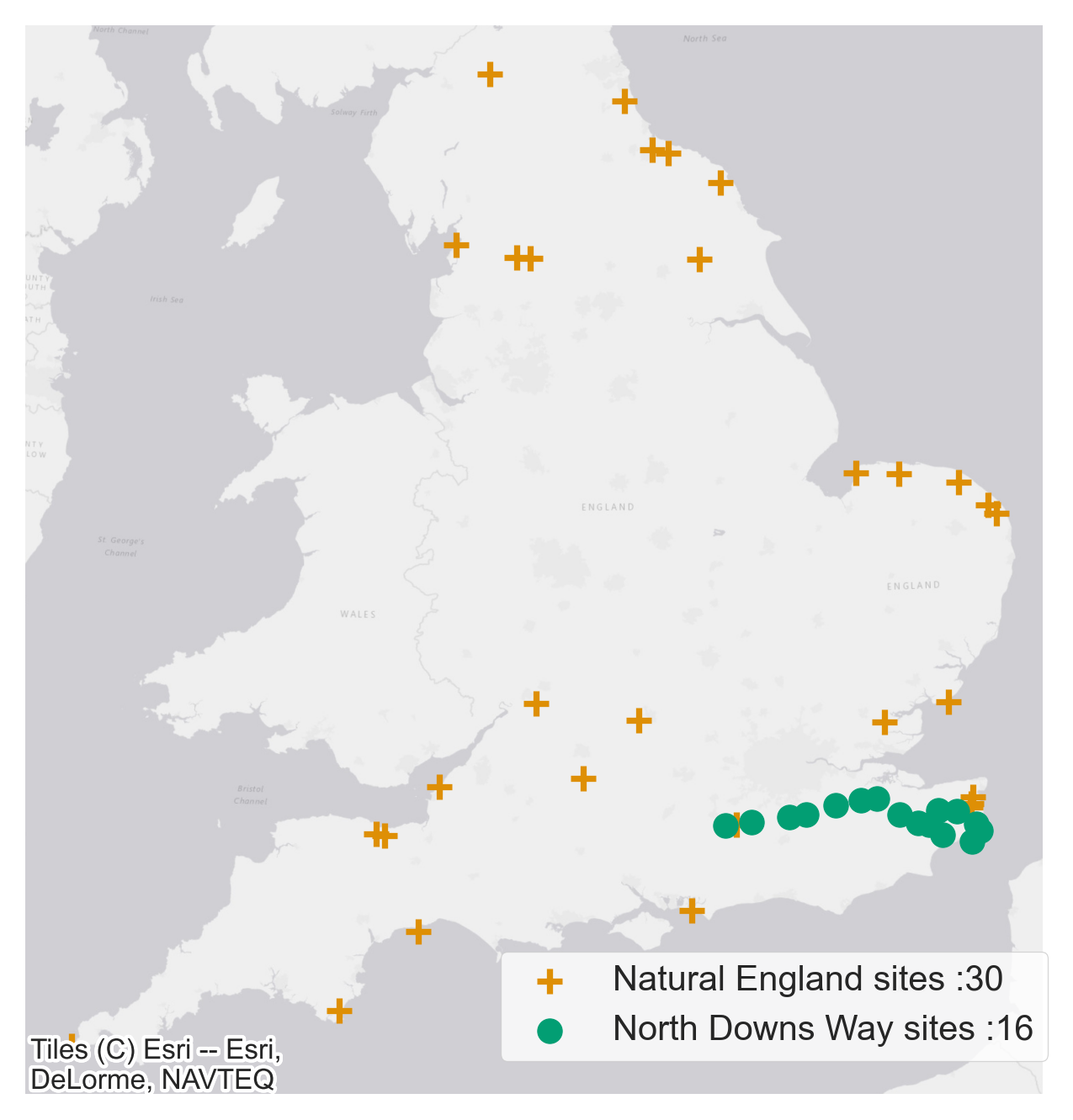
Strava Metro data:
Strava Metro provides controlled free access to the largest collection of human-powered transport information in the world. Metro aggregates, de-identifies and contextualizes Strava data and provides this data to public sector partners who promote active transport and lifestyles. Activity tracking and sharing apps, such as Strava, provide a growing source of data for mapping physical activities. However, the data is biased, generated from only app users.
To overcome this challenge, we recalibrated Strava activity data by integrating Strava Metro with other datasets on socioeconomic profile, land use characteristics, and weather conditions into our model to predict number of visitors in natural spaces.
Other relevant datasets:
We carried out an extensive literature review and engaged closely with Natural England subject matter experts on green infrastructure, access, and recreation, to identify additional datasets relevant for our investigation. This is summarised in Figure 3.
Figure 3: A list of different dynamic and static variables combined to develop a spatio-temporal model to estimate Monthly Average People Count.
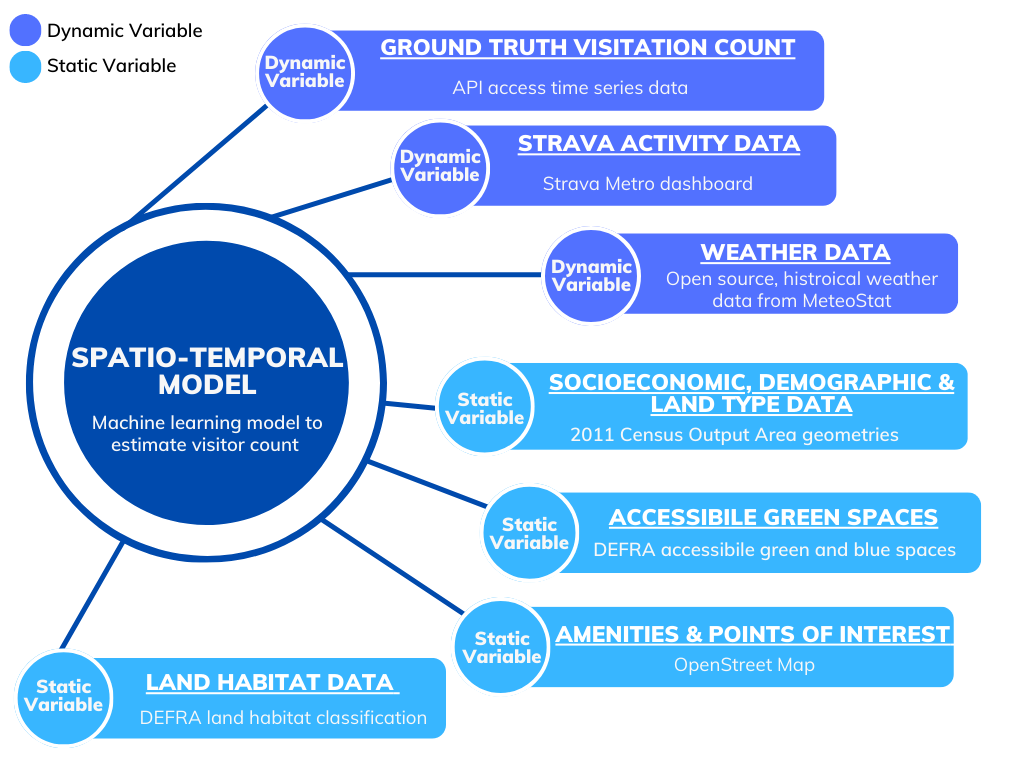
Model development
Our modelling approach involves estimating the monthly average people count (MAPC) to best replicate the visitor numbers from the automated people counters. The dataset used to train our model was created by extracting features from all datasets mentioned above in a five-kilometre area surrounding the location of the automated people counters. Five kilometres was selected based on evidence from the ORVal Insights project, suggesting that the probability of an individual visiting a natural space decreased substantially when the location was further than five kilometres away.
Some of the features extracted include Strava Metro data relating to the total number of journeys on foot, land and habitat classification data, socio-economic and demographic features, points of interest data and weather data. Using the data from this training dataset we created estimations of MAPC using an ensemble learning approach. The ensemble model combines the estimations of several machine learning models to improve generalisability and robustness when compared to a single model.
To evaluate and calibrate the estimations made by our model we compared the model outcome to the visitor numbers from the automated people counters data. As the inputs to the training dataset are available for a much wider areas than the automated people counters, we are not limited to making estimations only for the locations with people counters (after calibrating the model against the places where people counters are installed).
The model estimations can be generated for a diverse range of natural spaces across England. Full technical detail of our modelling approach, open-source code, sample data and a technical paper will be provided in the near future.
Figure 4 shows the performance of the model. The model estimation of MAPC for each site is aggregated to a regional level and compared to the observational automated people counter data. The model performance is better in regions with a higher number of training sites (locations monitored with automated people counters).
For example, model predictions in South East and South West regions are more similar to the Actual Count than the predictions in North West region. Across South East, South West and Yorkshire and the Humber regions the model predictions successfully capture annual seasonal trends present in the Actual Count data.
In Figure 5 we have shown the error in the predictions from the model and the actual people counter numbers for different regions confirming the model performance is better in regions with more representative training sites.
Figure 4:(a) Comparison of regional mean of Monthly Average People Count estimated by our model to the automated people counter data. (b) People counter sites grouped by UK Region.
(a)
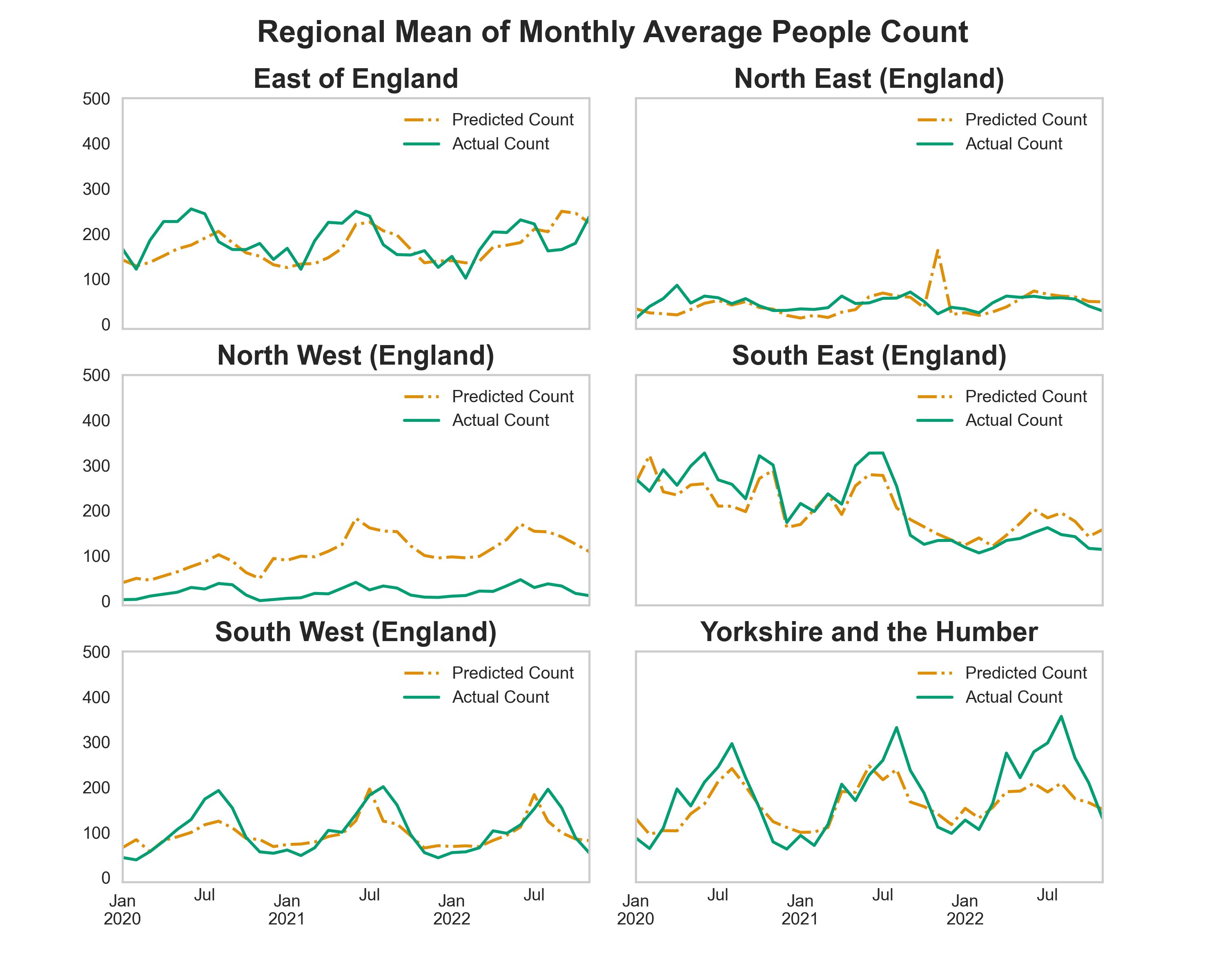
(b)
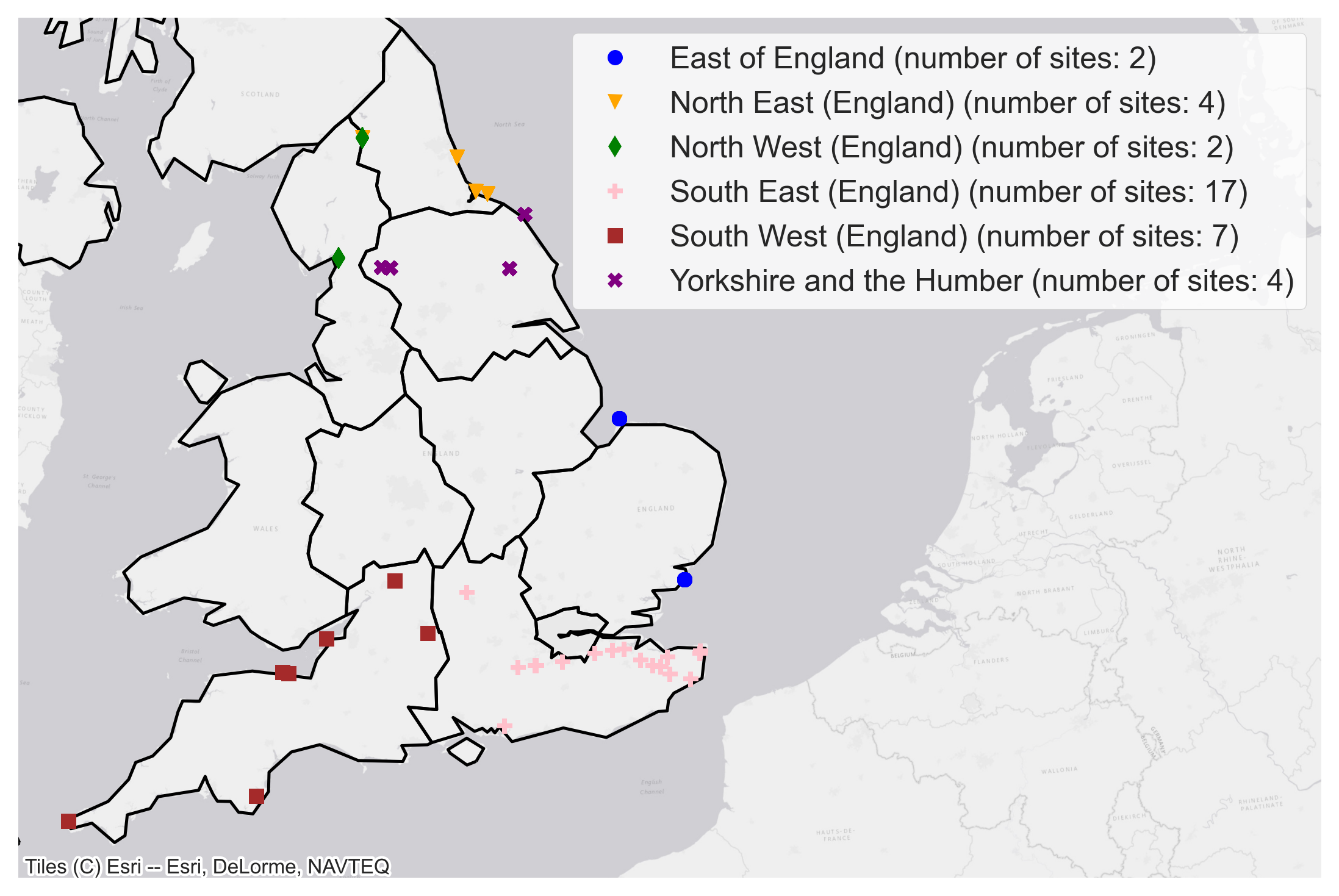
Figure 5: Error in prediction of machine learning model in comparison to the ground truth people count numbers. Calculated by Actual Count minus Predicted Count.
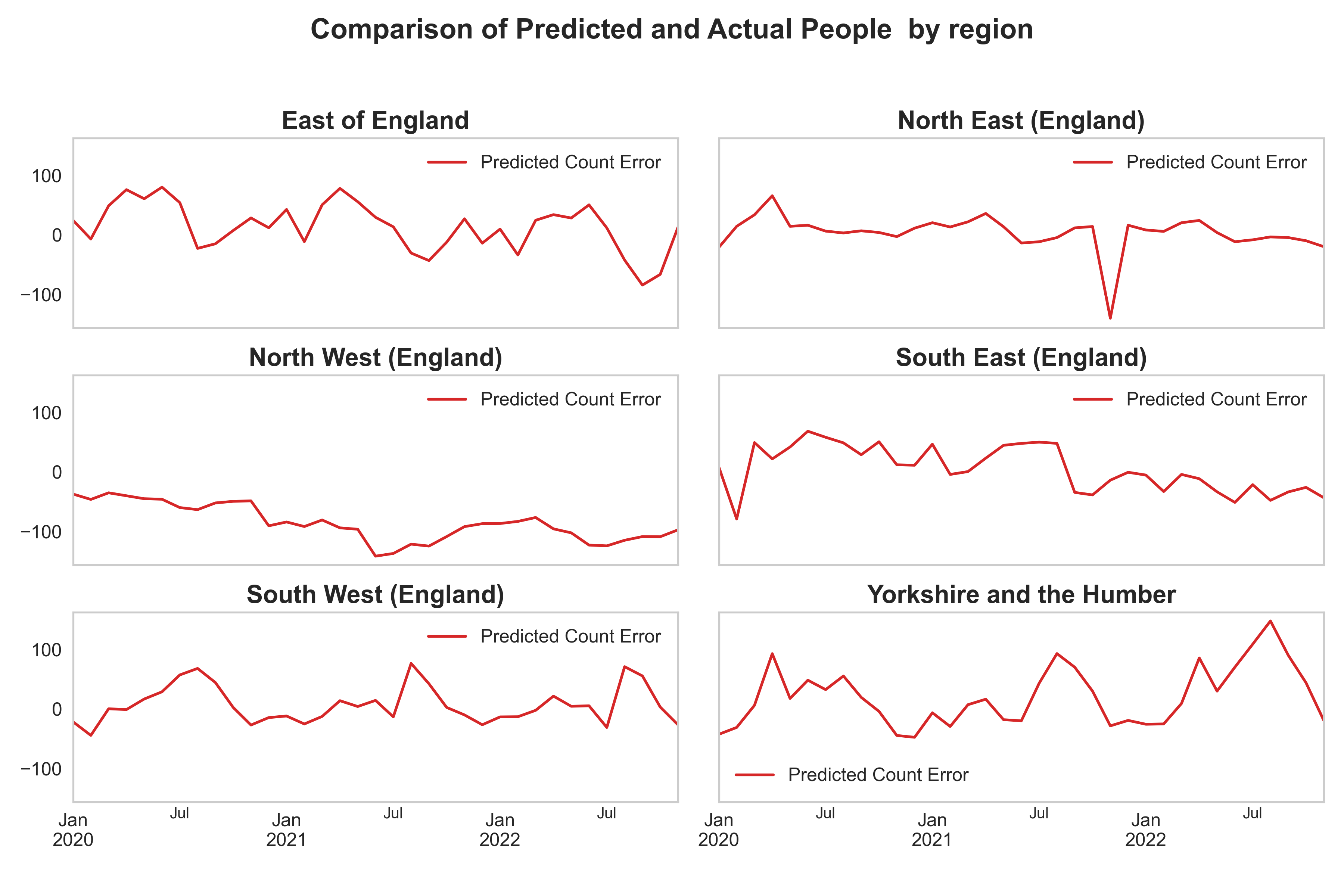
Limitations and Next Steps:
Model performance is strongly influenced by the availability of ground truth people counter data. In the next phase, we plan to work with a range of partners that maintain and can share people counter data to address this limitation.
Over the next 12 months plans are in place to use additional sources of people counter data to improve the model estimations and recalibration of Strava Metro data. The outputs will then be used to develop a new experimental statistic on visits to natural places.
Summary
This project has successfully developed proof of concept machine learning model that can estimate monthly average people count for a diverse range of natural sites across England.
The model developed has a higher spatial and temporal resolution than existing methods of measuring nature engagement and is also fully scalable to include more ground truth data as and when it becomes available. The approach is based entirely on crowdsourced and open datasets.
We intend to publish the code alongside a further technical paper and the analysis is also fully reproducible. The outputs can aid decision making and offer a low-cost alternative to existing methods such as automated people counters.
This approach has a wide range of potential uses. For example, measuring the use of new trails like the England Coastal Path or estimating visits to National Parks, accessible greenspace or nature reserves . It could also offer a model for measuring general activity levels in a range of other policy settings such as health, active travel, recreation, and tourism.
If you have any inquires about this project please contact: Tim Ashelford @ Tim.Ashelford@defra.gov.uk