Understanding the characteristics of high growth companies using non-traditional data sources
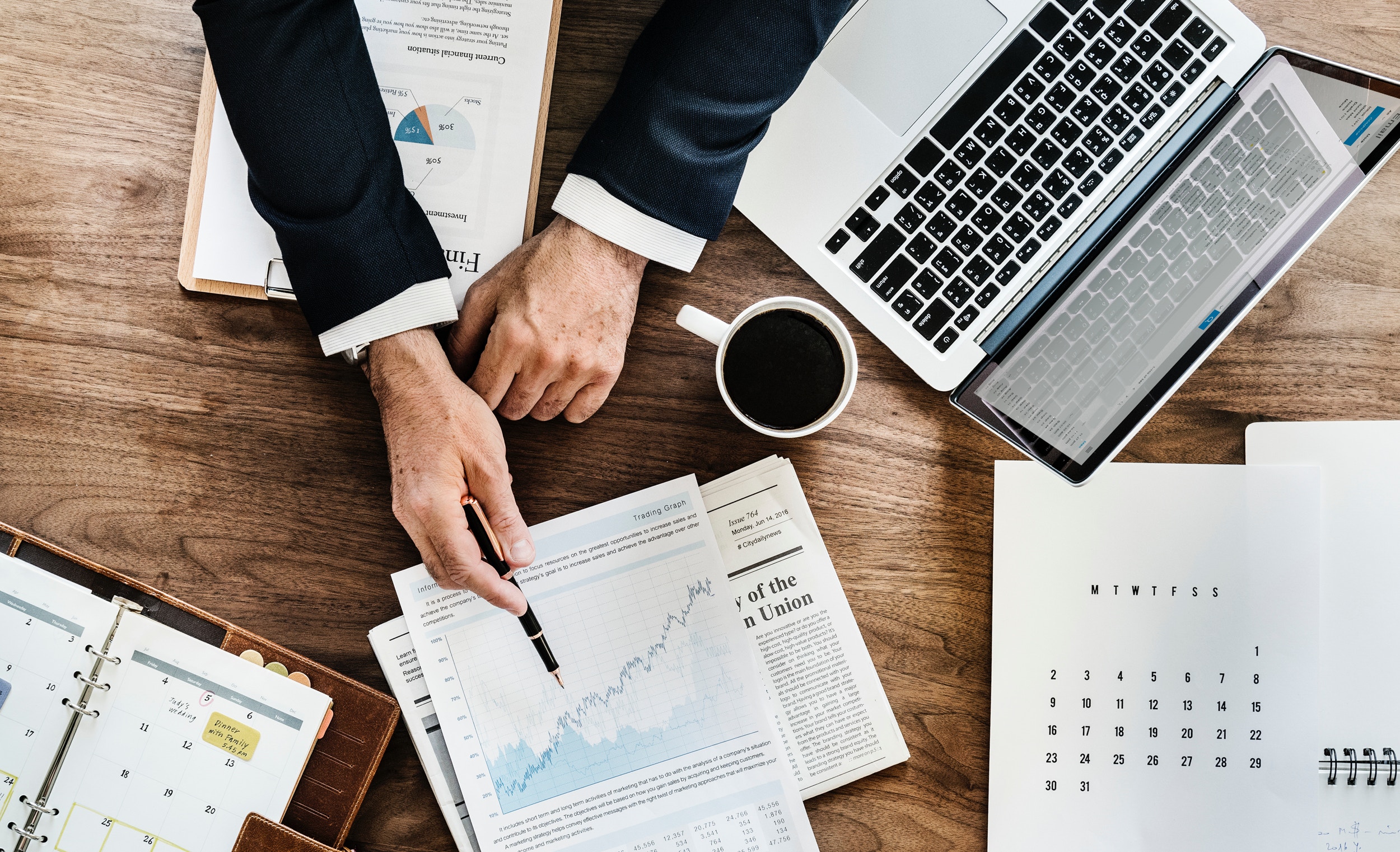
Updated: 17 October 2019
This report was originally published on 14 January 2019. Today, we have published an update exploring whether firms engaging in certain management practices were more likely to be classed as high-growth. Updates have been published in the following sections:
Section 2.8 Management and Expectations Survey dataset
Section 5 – Exploration of Management Practices
Section 6 – Conclusions
We would like to thank Data Science Campus apprentices Jonathan Rees and Evie Brown, who completed this follow-up work.
Introduction
High growth businesses drive economic growth in the UK. Predicting if a business has the potential to show high growth – or alternatively low performance – could be used to target where and how much people invest, where people choose to work and what support structures and policies are developed and put in place. Therefore, understanding the characteristics that may lead to companies showing high performance is an area of active research. Many of these research approaches tend to use more traditional datasets and methods. ‘Non-traditional data’ in this context broadly refers to data initially collected for a purpose other than statistics, research or administration, for example data collected about a company from the web. This report outlines work exploring how non-traditional data sources and data science methods can be combined with more conventional business data to help understand the characteristics and behaviours of high growth companies.
This project carried out by the Office for National Statistics (ONS) Data Science Campus (the Campus) is part of a wider Data Enabled Change Accelerator (DECA) project led by the Department for Business, Energy and Industrial Strategy (BEIS). The larger project aims to identify the characteristics of businesses with high growth potential using HMRC tax data. This report outlines work that combined business administrative data with non-traditional datasets, such as geographical features and websites data, with the objective of understanding if this adds insight into the key features that characterise companies with high growth. An exploration of the datasets and the features engineered from their variables is described along with an assessment of whether they contribute to understanding high growth.
The scope for the Campus does not include predicting which organisations are likely to achieve high growth. ONS is legally obliged not to identify individuals or individual companies in our outputs, so this work looks only at group characteristics. The Campus used algorithms in order to identify feature importance only, the work presented does not include how to predict high growth and the models were not tuned for this purpose.
In this project we have explored the potential for non-traditional data sources to add to our understanding of high-growth businesses and business sectors.
The understanding of what makes a company high growth is varied and complex and the work presented is used to illustrate the potential explanatory power of the datasets, but should not be taken as a full analysis in to this area.
1. Background
The definition of what constitutes a high growth company changes within both academia and industry, with each having its own benefits and drawbacks. The most commonly used definition is that of the Organisation for Economic Co-opertation and Development (OECD)-Eurostat (2007); which identifies high growth firms as “enterprises with average annualised growth in employees or turnover greater than 20 percent per annum, over a three-year period, and with more than 10 employees in the beginning of the observation period“. This is useful as it allows both longitudinal and cross sector comparisons, however, this measurement can be skewed with bias towards smaller and younger companies as these are likely to discover higher proportions of growth. Due to the relative ease of measurement and availability of data this was the definition used during this body of work.
The existing volume of literature examining high growth companies falls within two categories:
- quantitative studies assessing the economic contribution of high growth firms, seeking to identify their distinctive characteristics
- qualitative studies based on surveys of high growth firms concerned with identifying commonalities in their distinctive features as a means of explaining rapid growth. Few studies have investigated understanding the characteristics that allow the prediction of which firms would become high growth or when high growth episodes would occur. Instead they focused on the classification characteristics of existing high growth firms. Much of this research is based upon traditional industries and data sources. Although companies within the digital economy have relatively low coverage in this body of work, evidence suggests that high tech firms don’t show a higher rate of high growth companies compared with other sectors.
The huge impact that high growth companies have on employment growth was emphasised by a study, covering the period 2002 to 2008, that found that high growth companies represented around 6% of the total number of firms in the UK economy but created 54% of all net new jobs in the UK. The profile of these companies was like that found in many other studies of high growth firms – although young companies are more likely to achieve high growth, most high growth companies are small businesses consisting of fewer than 50 employees and are over five years old.
NESTA updated this research to cover the onset of the global financial crisis of 2008 to understand if the benefits of high growth on employment only occurred in times of prosperity. The study covered 2008-2010 and observed that the number of high growth companies was similar to the periods 2002-2005 and 2005-2008. High growth firms generated more than half of all new jobs created by firms with 10 or more employees, leading to the conclusion that high growth firms carry equal significance in periods of economic growth and periods of recession.
Many of the studies looking at the distinctive characteristics of high growth firms identify many areas of interest. One such of these being that of the entrepreneur behind the firm and founder related variables; such as the size of the management team behind the venture, their education and experience levels as well as their motivation were found to be important features in high growth firms. The networks of the directors and their relationships to other firms were also found to be important.
The geographical location of the firm was also found to be highly significant and potentially a very interesting area of study. Henrekson and Johansson discovered that the sector of the economy and the industry type is not a strong predictor of high growth, but the geographical location in the form of clustering is. It has been suggested that clustering increases the competitive environment of a locality, requiring firms to innovate in order to remain competitive – this in turn has beneficial effects on growth. The distribution of digital companies (PDF, 6.1MB) using travel to work areas has also revealed some geographical trends.
The demand for products or services can be enhanced when similar companies cluster together. A brand name recognition of the region and cluster can be generated and this can be particularly important for retail. In 2006 and 2008 Gilbert, McDougall and Audretsch found these firms have a greater ability to harness this reputational advantage than those not in a cluster, leading to increased growth performance. Geographical clusters provide greater access to both customers and to network opportunities with prospective business partners.
Some of the few areas of consensus are that high growth companies are not concentrated in any particular sector but are more common in sectors that are growing. Geographical location bears some importance, especially in the existence of clusters of competition. It is repeatedly found that fast growth is not a state that is consistent over time, instead it is cyclical in nature with companies experiencing waves of high growth followed by slower growth. Young companies in particular are prone to experiencing growth setbacks after a period of rapid growth.
2. Datasets
Data were sourced from three institutions; Glass AI, the Department of Business, Energy and Industrial Strategy (BEIS) and Ordnance Survey, in addition to using the Office for National Statistics (ONS) own interdepartmental register (IDBR). The datasets used are described in this section.
2.1 Ethics
All data collection and merging has been ethically reviewed within ONS as agreed by National Statistician’s Data Ethics Advisory Committee. The Glass AI web reading policy is outlined in Section 10.1.
2.2 Interdepartmental Business Register (IDBR)
The IDBR is a structured list of UK businesses. The two main sources of input are:
- Value Added Tax (VAT) system from HM Revenue and Customs (HMRC) (Customs)
- Pay As You Earn (PAYE) from HMRC (Revenue)
Additional input comes from Companies House, Dun and Bradstreet and the Office for National Statistics (ONS) business surveys. The IDBR covers 2.6 million businesses in all sectors of the UK economy, other than very small businesses (those without employees and with turnover below the tax threshold) and some non-profit making organisations.
Within the framework of this project the IDBR has been used to provide a definitive list of businesses and to link other datasets using the enterprise_id.
Table 1: Overview of the variables in the IDBR dataset
Variable | Description |
Enterprise_id | Unique identifier for a business |
Employees | Number of employees aged 16 years and over that a business pays from its payroll(s), in return for carrying out a full-time or part-time job or being in training |
Employment | Employees (as per above) also including Working Owners and Directors who are not paid via the PAYE |
SIC 2007 | Standard industrial classification of economic activities, 2007
|
ONS UK region | Which region of the UK a company is registered to, for example, Wales or the South West |
Ultfoc_gb | The ultimate parent of the business, if ‘GB’ this company is owned within Great Britain |
2.3 Glass AI
Glass AI is a large-scale artificial intelligence system that reads, interprets and monitors the open internet. The company is building a new research resource for social, economic and market analysis. So far, Glass AI has digitally mapped the UK economy, tracking any topic of interest across hundreds of millions of web pages, and over 1.5 million organisations. The data shared by Glass AI was based on a random sample of 30,000 UK active companies (company websites have been active/visited within a couple of months previous to the website reading) but excluding .org and org.uk sites (for example non-profit and government organisations). The data also included descriptions, sector classifications, mentions, news articles, job adverts and bios as detailed below.
2.3.1 Glass AI mentions data
Table 2: Overview of the variables in the Glass AI mentions dataset
Variable | Description |
id_organization | unique identifier for company (uniqueness based on website address) |
organization_name | Company name |
Website | Company website address |
organization_sector | Glass AI assigned sector, contains two parts – sector and subsector |
description | Company description extracted from website (e.g. from about me page) |
timestamp | Timestamp of data website reading |
topics | Glass AI derived main topics from the company website data |
organization_mention_out | Number of detected references made on organisation’s website to other organisations |
organization_mention_in | Number of detected mentions of references to organisation made on other websites |
people_mention_out | Number of detected references to organisations made in people’s biographies on organisation’s website |
people_mention_in | Number of detected organisation mentions out |
2.3.2 Glass AI news data
Table 3: Overview of the variables in the Glass AI news dataset
Variable | Description |
id_organization | unique identifier for company (uniqueness based on website address), an organisation can have multiple entries |
timestamp | Timestamp of data read that detected the news article |
title | Title of news articles published on the organisation’s website |
content | Full text of news articles published on the organisation’s website |
2.3.3 Glass AI jobs data
Table 4: Overview of variables in the Glass AI jobs data
Variable | Description |
id_organization | unique identifier for company (uniqueness based on website address), an organisation can have multiple entries |
timestamp | Timestamp of data website reading that detected the job advert |
job_title | Job title published on the organisation’s website |
job_description | Full text of job adverts published on the organisation’s website |
2.3.4 Glass AI bios data
Table 5: Overview of the variables in the Glass AI biography data
Variable | Description |
id_organization | unique identifier for company (uniqueness based on website address), an organisation can have multiple entries |
timestamp | Timestamp of web data reading that detected a change to the biography |
people_name | Name of person the biography relates to |
people_role | Job role of person the biography relates to |
people_bio | Full text of biographies published on the organisation’s website |
2.4 High growth flag
Department for Business, Energy and Industrial Strategy (BEIS) created a longitudinal dataset using the interdepartmental business register (IDBR) data and HM Revenue and Customs (HMRC) Value Added Tax (VAT) data. This was used to create the flag that identifies high growth companies that is used in this work. The assessment of high growth is based on the Organisation of Economic Co-operation and Development (OECD) definition of high growth in employees (compound 20% growth per year over a three year period). As the definition requires three years of data, the latest cohort of enterprises which could be assessed were from 2013. Therefore, the dataset was created from companies that were active in 2013 with 10 or more employees, with a flag indicating whether they had a high growth episode between 2013 and 2016 (that is, 72.8% growth over the three-year period). The variables that are contained within the dataset are given in table 6.
Table 6: Overview of the high growth flag dataset
Variable | Description |
enterprise_id | Unique identifier for a business, all organisations have a legal status of 1, 2, 3 or 4 in all the extracts of the IDBR used by BEIS |
extract_year | The year of the March IDBR extract which contained the data about the employees in 2013, for example, extract year 2017 relates to the IDBR data BEIS received at the end of March 2017 |
outcome_high_growth01 | Whether the enterprise was classified as high growth at the end of the period as follows:
|
2.5 Retail clusters
Data was produced by Ordnance Survey (OS) that allows identification of whether a business is located within a retail cluster. This can lead to insights on whether high growth companies cluster in retail areas. To qualify, a cluster must contain at least three properties classified as retail in the OS AddressBase product. This includes businesses such as banks, markets, petrol stations, pubs, restaurants and fast food outlets as well as shops. These properties must be less than 70 metres apart from each other.
The Campus thank OS for sharing the data with us. It allowed an exploration of whether location within a retail cluster is an indicator of high growth. The Campus selected six local authorities to analyse based on the data available at the time: Basingstoke and Dean, Birmingham, Glasgow, Warwick, County Durham and Neath Port Talbot. These six districts were chosen as a sample to cover a range of different local authorities with a mix of rural-urban indicators from across the UK.
The data used contained retail clusters that spatially covered the six local authority district boundaries. The geography describing the extent of a retail cluster was provided as a multi polygon geometry in a GeoJSON format using the WGS84 (ESPG: 4326) spatial projection. Each retail cluster has an associated ID and an accompanying postcode lookup file. Figure 1 shows that a postcode unit is included in the lookup where the postcode polygon intersects the multi polygon geometry of the retail cluster.
Figure 1: Retail cluster (in blue) shown for near the centre or Birmingham. Where a postcode region (the postcode centre is shown by the grey circle) intersects a retail cluster (blue) polygon then it is included in the postcode lookup.
2.6 Travel to work areas
In addition to retail clusters it is interesting to investigate if labour market can affect the growth of companies. The ONS Travel to Work Areas (TTWAs) data are geographies created to approximate labour market areas. They are derived to reflect self-contained areas in which most people live and work. The current criteria for defining TTWAs are that at least 75% of the area’s resident workforce work in the area and at least 75% of the people who work in the area also live in the area. The area must also have an economically active population of at least 3,500. There are 228 TTWAs in the UK (as estimated using Census 2011 data). If a postcode of a property is known then its appropriate TTWA can be determined through the ONS Postcode lookup dataset.
2.7 ONS postcode lookup
The Office for National Statistics Postcode Lookup (NSPL) data for the UK is a freely available dataset that relates current postcodes to a range of current statutory administrative, electoral, health and other statistical geographies via “best-fit” allocation from the 2011 Census output areas. It supports the production of area based statistics from data that includes a postcode.
2.8 Management and Expectations Survey
The Management and Expectation Survey (MES) is a questionnaire run jointly by the Office for National Statistics (ONS) and Economic Statistics Centre of Excellence (ESCoE). The survey’s aim is to gather information on businesses’ management practices and future expectations on turnover, employment, expenditure and capital investment growth.
Table 7: Overview of the main variables in the MES dataset
Variable | Description |
entref | Enterprise-ID number unique for each business and consistent between the BEIS dataset and MES survey |
FormStatus | Details the response status of each business. For instance; Clean data, Voluntary Refusal, Awaiting return |
extract_year | Year which business was reviewed for high growth. Taken from BEIS High growth dataset |
location | UK NUTS location of the business |
emp_2016 | The total number of employees on the pay roll of the business on 5th April 2016 |
turn_2016 | The turnover of the business in 2016 |
q1-q20 | List of questions in the MES survey. Some questions have been split into parts A and B, Where Part A refers to managers, and Part B refers to non-managers |
gdp (nine potential options, ranging from ‘strong decline’ to ‘strong increase’) | The change in UK GDP in 2017 and 2018 predicted by the business |
This survey is not mandatory for businesses, and a large proportion of businesses did not respond to the survey or responded with unusable data. This is shown in Table 8. There were also variations in the number of businesses that answered each question.
Table 8: Reasons for missing submissions, as a percentage (%) of the merged datasets
Form Status | % of businesses |
Awaiting response from business | 56.1 |
Voluntary refusal of survey | 3.6 |
Other | 2.2 |
Useable data | 38.1 |
2.9 Limitations of the data
A consideration to make when combining these datasets is the timing (reference period) of the information being compared. We do not know whether the reference period of the IDBR information is the same as that of the Glass AI data. Each source has a differing frequency and regularity. For example, the IDBR data is updated with samples regularly and combined from other sources, while the TTWAs are updated from each 10-year census. The web data read by Glass AI is perhaps the most complex. Data timestamps refer to the date the item was first read or a change was detected. The item could have been posted a long time before it was first read and it is impossible to detect which data items come from websites that are regularly updated and well maintained and which are static and possibly out of date.
In relation to this project it hasn’t been possible to restrict the websites data to only items from before or during the high growth period. All available time periods have been included.
3. Identifying the features of high growth firms
Data was loaded, merged and analysed using Python using the environment outlined in Section 10.3. The interdepartmental business register (IDBR) and the Department for Business, Energy and Industrial Strategy (BEIS) flag were merged on the unique enterprise ID. This led to a dataset of just under 6,600 matched records (see Section 10.2). Further merging with the Glass AI data led to a final dataset of just over 5,500 records with information about whether a company was classed as high growth. Of these 8.6% of companies were high growth.
Figure 2: Summary of IDBR and Glass AI combined dataset
3.1 Understanding features using prediction models
The Glass AI websites data was combined with the IDBR data to understand if it could improve the ability to understand the characteristics of businesses with high growth potential. In order to find these characteristics we used the same techniques as for classification prediction, although predicting individual businesses was not the aim of this exercise.
Five different supervised learning classification models were assessed to understand how well they could predict high growth companies. These were logistic regression, support vector machine (SVM), decision tree and forest type approaches (see Table 19 in Section 10.4). The models were first applied to the IBDR data with the BEIS high growth indicator as the target using different subsets of features (see Table 9). This provided a baseline. The modelling was then repeated with the addition of the Glass AI data to see if an improvement in the model performance was observed.
The dataset was unbalanced with the high growth firms (HGF) making up less than 9% of the records. Machine learning algorithms have trouble learning when one class dominates the other, as they can learn to class all inputs as the majority class and still achieve high scores. Several different techniques were used to balance the data. As the dataset size is relatively small, under sampling of the standard companies was avoided. Instead focus was on oversampling and synthetic data techniques. These were the built-in class_weight balanced option within SciKit Learn and the Random Naïve Sampling and Synthetic Minority Oversampling Technique (SMOTE) methods from the imbalanced_learn package. Four variants of SMOTE were applied – regular, borderline1, borderline2, SVM – alongside the adaptive synthetic (ADASYN) sampling approach (see Section 10.5.1). Data was split into train and test sets using a 30% ratio before applying the models, and the models were assessed by calculating the Matthews correlation coefficient (MCC), specificity and the rate of standard growth companies correctly identified. A number of feature sets were used during model training, see Table 9 for details.
Table 9: Summary of the two main feature sets used for model training
Feature | Model Feature Set 1 | Model Feature Set 2 |
Employees | x | x |
Employees_d | x | x |
SIC 2008 (2 digit divisional level) | x (not used for Glass AI) | x (not used for Glass AI) |
ONS UK Region | x | |
Ultfoc_gb | x | x |
TTWA | x | |
People mentions in | +Glass AI | +Glass AI |
People mentions out | +Glass AI | +Glass AI |
Organisation mentions in | +Glass AI | +Glass AI |
Organisation mentions out | +Glass AI | +Glass AI |
Glass AI main Sector | +Glass AI | +Glass AI |
Features marked as Glass AI were used when testing the effect of adding websites’ data.
A number of features were engineered to use in the models. The employees_d feature was created as the difference between the number of employees aged over 16 years and paid from the business payroll (employees) and the number of employees including working owners, consultants and directors not paid via PAYE. This is a proxy for indicating the number of consultants and directors a business has. The Ultfoc_gb is a flag that indicates whether the owner of a business is based in the UK or abroad.
SICs come from business surveys, and are consistent with SIC2007 Standard industrial classification of economic activities (SIC) Code 2007. The 88 two-digit code representing SIC division was used as a feature. This was included when using just the IDBR data. When the Glass AI data was included this was dropped and the Glass AI sector 1 (consisting of 12 categories) was used instead. The Glass AI sector potentially reflect industry sectors not defined in SIC 2007 such as digital marketing. A correlation between the two was observed and so the two were not used together in the models.
Without the Glass AI data, the tree-based models provided the best performance (Table 10). The models in general are quite poor, only marginally providing an improvement over random classification in terms of predicting high growth companies. In general Gradient Boosted Classifier (GBC) and Random Forest with Naïve Random Oversampling performed the best for a number of different measures. However, care must be taken when interpreting the models. A high sensitivity (ability to find all high growth companies)[1] and precision (accuracy of predicting high growth companies) are required, but whilst minimising the number of firms incorrectly predicted as high growth (false positives, FP). Additionally, the ability to correctly identify companies that will not be high growth (true negatives, TN) may also be useful in targeting businesses with potential to grow. For example, if you can immediately discount say 50% of a population as never going to be high growth, it allows us to focus resource on the remaining 50% that may contain businesses that could have potential. The end application is important in fully interpreting and fine-tuning models, and in this study we are concerned only with characteristics.
The Matthews correlation coefficient (MCC) is the main quality measure used to assess the models. It is a balanced measure used for binary classification and can be used for unbalanced datasets. It is a correlation coefficient between the observed and predicted binary classifications and returns a value between -1 and +1. A coefficient of +1 represents a perfect prediction, 0 indicates a prediction that is no better than random and -1 indicates total disagreement between prediction and observation. It was found to be the best method to assess the ability of the model to have a high sensitivity, specificity and precision – maximising the proportion of high growth companies identified whilst minimising the number of standard growth firms incorrectly classified as high growth.
Table 10: Results averaged over five-fold cross validation runs of GBC using naïve random oversampling with and without Glass AI for feature set 1 and 2
Featureset 1 | Featureset 2 | ||||
Quality measure | Description | IDBR only | IDBR + Glass AI | IDBR only | IDBR + Glass AI |
MCC | Effectively the % of the total population identified as never going to be high growth | 0.185 | 0.175 | 0.193 | 0.171 |
% True negatives (TN) identified | 58.3 | 64.0 | 59.1 | 61.2 | |
% of HG companies identified (specificity) | 74.5 | 65.9 | 74.5 |
68.4 |
An example of the results from the GBC model with Naïve random oversampling for two different feature sets is shown in Table 10. This shows a summary of the results from GBC model with the feature sets outlined in Table 9. The model was run using a five-fold cross-validation and the average results are shown. The feature sets provide a poor input for classifying a company as high or standard growth, with MCC scores of under +0.2. Tuning the model and oversampling methods did improve the number of high growth firms predicted but this is at the expense of predicting a high number of false positives, showing no improvement in the MCC and overall usefulness of the models.
Figure 3: Mathews Correlation Coefficient scores for IDBR only and IDBR + Glass AI mentions data using GBC model and naive random oversampling
There is no improvement in the model scores when adding Glass AI data.
In order to assess the addition of web “mentions” data the models were rerun using the Glass AI data as extra features. This included Glass AI’s own sector category instead of Standard Industrial Classification (SIC) code and number of mentions in and out for people and organisations. For both feature sets a small drop in MCC was observed when the Glass AI was added (Figure 3). The Glass AI organisation and people “mentions” data did appear as part of the top five features for GBC as shown in Figure 3, showing they are contributing a higher influence in the models compared with some of the more traditional variables such as SIC division code and region. These “mentions” features are a proxy measure of the connections of the companies online and suggest that high growth firms are more likely to have a bigger network. The Glass AI sector type – which could be an alternative version of the 2007 SIC code – did not appear as one of the top five main features. When repeated using the SIC code instead of the Glass AI sector the models did not change.
Adding other Glass AI dataset features to the model was performed but the further merging reduced the number of data points available for fitting with the available data samples. For example, adding the bios and job count reduces the data to under 500 data points.
Figure 4: Main five features from GBC model using IDBR only and IDBR + Glass AI Data
Plots on the left show the main features when using just the IDBR data, and plots on the right show IDBR + Glass AI. The top row use feature set one and the bottom feature set two. The number of employees and the difference between the number of employees aged over 16 years and paid from the business payroll (employees) and the number of employees including working owners, consultants and directors not paid via PAYE (employees_D) are main features for IDBR data only, along with SIC divisions. The London area and London TTWA also feature. When Glass AI data is added we see the people and organisation mentions in and out becoming main features
[1] Sensitivity is the proportion of 1s correctly classified, specificity is the proportion of 0s correctly classified and the precision is the proportion of predicted 1s that are actually 1s. Getting a good balance of all of these scores is important for imbalanced data sets and which are more important depends on the application – for example, for detecting a rare aggressive cancer it is more preferable to correctly detect as many positive cases as possible so a high sensitivity is important. However, incorrectly diagnosing a negative as a positive case (low precision) would result in unnecessary treatment.
3.2 Topic analysis
The different topics and styles of speech used by companies on their websites was also of interest. Applying natural language processing (NLP) and unsupervised learning on free text data recovered from company websites it was possible to gain insight into characteristics in style of speech by different companies. Specifically, it was investigated if high growth companies use different words and topics compared with standard growth companies. The features investigated were the company description, news articles, job adverts and employee biographies.
Data was pre-processed to remove special characters before further processing converted the text into lowercase, tokenised, removal of stop words and stemming. Data was then analysed to identify main topics using non-negative matrix factorisation (NMF). NMF is a popular linear algebra model, often combined with term frequency-inverse document frequency (TF-IDF) for topic analysis. In simple terms, it takes a large body of text as an input and automatically detects words that appear together and groups them into topics. The assumption is that each document contains a finite number of topics, and each topic uses only a small set of words frequently.
TF-IDF and NMF were applied to the datasets for 2 to 10 topics. Each data record was then analysed and assigned to the most likely topic. The proportion of records for high growth and standard companies were calculated for each topic and then compared. Small differences were seen in the types of words and topics that high growth firms used. A summary of the observations is shown in Figure 5. It is observed that high growth companies tend to discuss general overview terms such as team and management more than specific terms like tax, law and manufacturing. This is regardless of whether they are describing the organisation, describing their people roles and biographies or in the news and jobs they post. This is an interesting first insight into the language used by businesses in their websites and could suggest that high growth companies are more interested in people and processes rather than specific tools or terms. Caution should be exercised since the dataset is small for this type of analysis and a larger dataset is required before further investigation and analysis. An area of interest is understanding if different business sectors use different terms and topics.
Figure 5: Summary of main words used by high growth companies for different free text collected from their websites
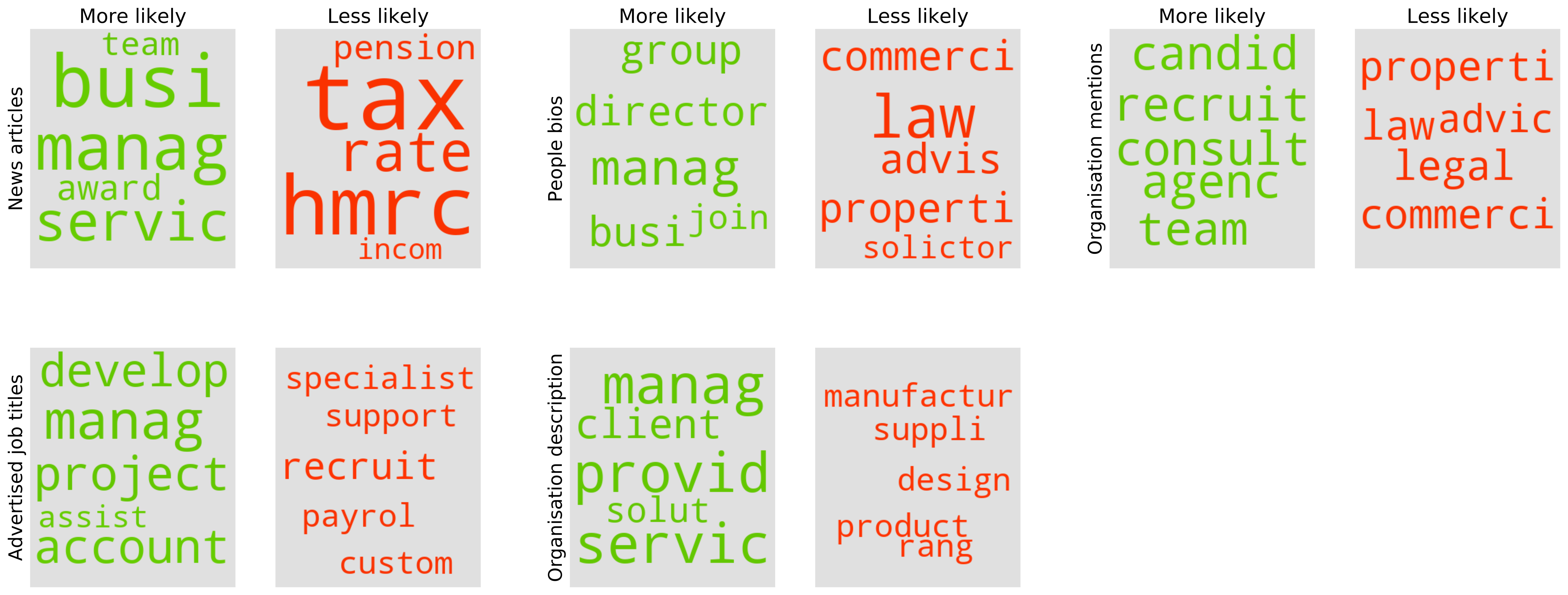
Text in green is more likely to be mentioned by high growth firms, whilst text in red is less likely to be mentioned for the different free text entries.
4. Geographical analysis
The location of a company could also be a characteristic that could influence whether a business will become high growth. The addition of the Office for National Statistics (ONS) postcode look up data allowed extra geographical data for businesses to be included. This includes district code and 2011 travel to work areas (TTWA). When merged with existing datasets that contains the company postcode several geographical features could be explored and linked to other datasets, allowing investigation to see if high growth firms were more likely to occur in certain areas or in certain clusters.
Merging the ONS postcode data with the enterprise postcode data yields a data set of 194,300 companies, 13,102 (6.7%) of which are classed as high growth.
Figure 6: Summary of IDBR and ONS Postcode Lookup data
4.1.1 Company distribution by region
The distribution of companies by region for the UK is shown in the left-hand part of figure 7. We observe that the South East and London dominate, both containing over 20% of the observed companies. The right-hand figure shows the distribution of high growth companies by region. We observe that London dominates this and has a higher concentration than the South East. London is the prime location for high growth businesses.
Figure 7: Distribution of all companies (left) and high growth companies (right) by region
4.1.2 Company distribution by districts
To understand the spatial distribution outlined in Figure 7 and to prepare for further analysis the distribution of companies by local authority district boundaries was investigated. A wide distribution of companies is observed across the districts. As expected the districts within large urban areas dominate in terms of the total number of companies. However, the rate of high growth companies varies within these regions from a high of 14.4% in Hackney (Table 12) to 2.4% in North Dorset. Ranking districts by the rate of high growth companies indicate that high growth businesses are more likely to be based in London districts. Some of this London dominance may be due to a head office effect – at enterprise level, firms likely to report via their head office, which is likely to be London, even though work is carried out elsewhere.
Table 11: Districts with the highest number of companies
% | ||||
District code | District name | No of companies | No of high growth companies | High growth rate |
E09000033 | Westminster | 4,200 | 458 | 9.8 |
E08000025 | Birmingham | 2,507 | 146 | 5.5 |
E09000001 | City of London | 2,154 | 323 | 13.0 |
E08000035 | Leeds | 2,182 | 202 | 8.4 |
E09000007 | Camden | 1,871 | 238 | 11.2 |
When ranked by highest rate of high growth companies we observe the London districts dominating the top five.
Table 12: Districts with the highest rate of high growth companies
% | ||||
District code | District name | No of companies | No of high growth companies | High growth rate |
E09000012 | Hackney | 811 | 117 | 14.4 |
E09000001 | City of London | 2,386 | 323 | 13.0 |
E09000028 | Southwark | 1,278 | 160 | 12.5 |
E09000019 | Islington | 1,275 | 158 | 12.4 |
E09000013 | Hammersmith and Fulham | 819 | 95 | 11.6 |
4.1.3 Company distribution by travel to work area
Instead of local authority boundaries it is interesting to see whether the density of working population, as indicated by TTWAs, can affect the location of companies. Using (TTWAs) (see Section 2.6), the distribution of companies and high growth companies was established. A high proportion of companies fell into the London (E3000234) TTWA. This had 26,241 companies, representing 14.9% of the observed UK companies. Of these companies, 2,652 were high growth, representing 9.2% of the companies in London and 20% of all observed high growth companies in the UK. There was a large drop off to the second largest TTWA in terms of company numbers. Manchester had just under 8,000 companies, 560 of which were high growth. This represents 4.1% of UK companies and 4.3% of all high growth companies. At the other end of the scale six TTWAs were observed with fewer than 30 companies, four of which were seen without any high growth companies (Wick, Campbeltown, Golspie and Brora and Channel Islands).
This again suggests that London dominates the UK in terms of the number of companies and the number and rate of high growth firms. As expected a wide distribution of companies and high growth companies is seen across the UK, with London dominating both.
Figure 8: Top 15 TTWA ranked by number of companies (top) and by rate of total observed high growth companies (bottom)
4.2 Company location within retail clusters
Due to the size and complexity of calculating the retail clusters, the data used includes retail clusters that spatially covers six local authority district boundaries. These six districts were chosen as a sample to cover a range of different local authorities across the UK. These local authority districts were: Basingstoke and Dean, Birmingham, Glasgow, Warwick, County Durham and Neath Port Talbot. These spanned a range of different city and mixed urban and rural districts from across the UK and fall into several groups
- urban city centre areas such as Birmingham and Glasgow, characterised by a large central retail cluster and many smaller retail clusters radiating out into the inner city and suburb areas
- more rural and urban mixed districts such as Basingstoke and Dean, Warwick and Neath Port Talbot, characterised by a number of small central retail areas and a sparse number of smaller clusters
- less sparse urban and rural mix such as County Durham that comprise of a number of neighbouring towns and villages, each with a number of central retail clusters
Table 13: Number and area of retail clusters in the seven different districts
km2 | |||
District | Number of retail clusters | Number of properties in a retail cluster | Total area of retail clusters |
Basingstoke and Dean | 43 | 737 | 1.51 |
Birmingham | 416 | 11,591 | 7.94 |
Neath Port Talbot | 56 | 1,189 | 0.45 |
County Durham | 180 | 4,430 | 1.98 |
Glasgow | 283 | 8,531 | 14.08 |
Warwick | 44 | 1,483 | 0.49 |
Figure 9: Retail clusters for the centre of Birmingham (top) and Basingstoke and Dean (bottom) showing the difference between the urban city districts and the more rural and urban districts
Reviewing the distributions of the number of addresses in a retail cluster and the area of retail clusters in the different districts, a distinct grouping between the city or more urban areas and the more rural districts emerges. The city and urban districts had a wider range in terms of the number and area of clusters. The city centre districts showed a larger single cluster representing the central shopping region. The rural areas showed a small number of larger clusters but the overall distribution showed a smaller number of clusters with fewer addresses and a smaller area.
Figure 10: Distributions for number of retail addresses in a cluster (top) and the area of a retail cluster (bottom) for the six districts
This OS retail cluster sample data was merged with other datasets and filtered to only include companies within the six districts. This was then analysed to see the proportion of high growth firms in and outside of retail clusters.
Figure 11: Summary of IDBR and location datasets
There were 6,940 companies matched to the BEIS high growth indicator. Of these 6.0% were high growth. In the districts covered by the OS data 17.5% of all companies were in a retail cluster (Table 14). A higher percentage of all the high growth companies were observed to be in a retail cluster – 22.7% of all high growth companies were located in a retail cluster compared with 17.2% of standard firms. 7.8% of companies located in a retail cluster were high growth, compared with 5.7% of companies located outside a cluster (Figure 12).
Table 14: Summary of high growth companies in and out of retail clusters in six districts of the UK
% | |||
Standard growth | High growth | Rate of high growth | |
In a cluster | 1,121 | 95 | 7.8 |
Not in a cluster | 5,400 | 324 | 5.7 |
Rate of company growth type located in a cluster (%) | 17.2 | 22.7 |
Figure 12: The rate of companies in a cluster by growth type (Top) and the rate of high growth companies in and out of a cluster (Bottom)
There is a higher rate of high growth companies in a retail cluster compared with standard growth.
Examining each district separately reveals large differences between the conurbation, city and more urban districts compared with the more rural and urban districts (Table 15). There is a large range of companies observed across the districts, but all districts had between 5.1 and 7.6% of their companies classed as high growth (Figure 13). The rate of companies observed to lie within a cluster varied from 2.8 to 28.3% (Figure 13), with the proportion of companies in a cluster that are high growth ranged from 0 to 9.4% (Figure 14). The rate of all high growth firms located in a cluster varied from 0 to 33.3%.
Table 15: Number of companies in each district and the number within a retail cluster
% | % | % | % | % | ||
Name | No companies in District | Rate of HG companies | Rate of all companies in a cluster | Rate of a cluster that is HG | Rate of all HG companies in a cluster | Rate of all Std companies in a cluster |
Birmingham | 2654 | 5.5 | 16.5 | 8.4 | 25.3 | 16.0 |
Glasgow | 1828 | 7.1 | 28.3 | 8.3 | 33.3 | 27.8 |
County Durham | 1109 | 5.1 | 7.7 | 9.4 | 14.3 | 7.3 |
Warwick | 536 | 6.2 | 28.2 | 4.0 | 18.2 | 28.8 |
Basingstoke & Dean | 535 | 6.4 | 2.8 | 6.7 | 2.9 | 2.8 |
Neath Port Talbot | 278 | 7.6 | 2.9 | 0 | 0 | 3.1 |
Figure 13: Rate of high growth firms by district
Figure 14: Rate of companies located in a retail cluster for each district
Birmingham, Glasgow and County Durham showed a higher rate of high growth companies located in a retail cluster than standard growth companies. They also showed higher rates of their high growth companies located in a cluster compared with out of a cluster (Figure 15). For Warwick, Basingstoke and Dean and Neath Port Talbot almost the opposite was observed.
Figure 15: Rate of companies located within a retail cluster for each district by growth type (left) and rate of high growth companies located in and out of retail clusters for each district (right)
Figure 16 shows the difference between the rate of standard growth and high growth companies located within each district. Positive difference shows a higher proportion of high growth companies compared to standard growth, while a negative difference indicates that the standard growth companies dominate. We observe that the larger urban city centres have a higher rate compared with the smaller city of Warwick. The interesting case here is County Durham that showed a high rate of high growth companies in retail clusters despite having a relatively lower urban index compared with the major city centres.
Figure 16: Difference between high growth and standard growth rates within retails clusters for the six districts
An urban or rural classification for each retail cluster would be of interest to extend this work. An indicator of the type of retail cluster would also be of interest. For example, does a cluster comprise of predominately service type businesses such as pubs, restaurants and fast-food outlets or is it primarily shops and does this affect the likelihood of a high growth company locating within that cluster. OS are developing and expanding this geographical dataset and the work should be repeated using these newer products once they are released.
To investigate the influence of urban and rural setting in more depth the merged interdepartmental business register (IDBR) and Office for National Statistics (ONS) postcode data was analysed to understand the distribution of high growth companies by the rural urban classification as defined by ONS using the 2011 census. Using the business postcode, it was possible to obtain a rural urban classification based on population and dwelling density – if businesses are located in an output area with a population of over 10,000 they are classed as urban, otherwise they are classed as rural. The urban categories are allocated into major conurbation, minor conurbation or city and town. Rural categories are allocated as town and fringe, village or hamlet and isolated dwelling based on the population density profile. There is further subdivision into sparse and not sparse based on the density profiles of surrounding areas.
Businesses that did fall into these classifications were neglected in this analysis. The rate of standard growth businesses in each category was compared with the rate of high growth. We observe that high growth companies are more likely to be found in major conurbations and less likely to be in cities and towns, providing further evidence that high growth businesses are more likely to be found in larger city regions.
Figure 17: The rate of standard and high growth companies for 2011 rural urban classification index
5. Management Practices
The Management and Expectations Survey (MES) asks questions on organisational structure and management practices, such as “how quickly is under-performance of employees addressed” or “how many key performance indicators are monitored”. The questionnaire originated in McKinsey, and has been used by researchers and for surveys carried out by other National Statistics Institutes (NSI). The Office for National Statistics (ONS) Management and Expectations Survey is based on these other surveys.
We explored the relationship between management practices and high growth by merging the responses from the Management and Expectations Survey (MES) with the Department for Business, Energy and Industrial Strategy (BEIS) high growth flag.
Matching the two sources results in a data set of 18,973 businesses, of which 9.9% were high growth.
Figure 18: Summary of BEIS high growth and MES
The BEIS high growth firm flag refers to business growth between the years 2013 to 2016, while the MES survey focussed only on the year 2016. Because of this, we cannot say from this work whether any particular management practices result in a business with a high growth rate. We can only say that businesses that engage in certain business practices were more likely to be classed as high growth from 2013 to 2016.
Using the merged dataset, we also grouped businesses together by employment size. 9.4% of small and medium enterprises (with less than 250 employees) have been high growth, whereas 10.5% of large businesses (with 250 employees or more) have been high growth.
This section describes MES questions relating to business characteristics, targets and employment practices that showed the biggest difference between high growth and regular businesses where as questions regarding key performance indicators and production delivery showed the smallest difference. For example, there was a 9% difference between high growth and regular businesses giving out performance bonuses to other staff members based on a target (employment practices). However, there was only a difference of 0.4% between high growth businesses and regular businesses who had staff reviewing key performance indicators.
5.1 Education
An area of interest is how the education and experience of directors is related to the growth of a business. Responses to the MES indicate high growth companies were more likely to have at least one employee with a degree compared to regular businesses. 86% of high growth businesses employed at least one manager with a degree compared to 80% of regular businesses and 83% of high growth businesses had at least one other staff member with a degree compared to 75% of regular businesses.
Figure 19 shows that as the proportion of managers with a degree increases the rate of high growth businesses also increases. However, having all managers with a degree shows a drop in the rate of high growth.
Figure 19: Variance from average high growth rate for the proportion of employees with a degree
5.2 Targets
Focusing on production targets reveals that high growth businesses were both more likely to set at least one production target and have employees aware of them. Table 16 shows that 90% of high growth businesses set at least one production target compared to 86% of regular businesses. All the businesses that set production targets had at least one manager aware of them. Awareness from non-managerial staff was lower. Only 83% of high growth businesses had at least one other non-managerial member of staff aware of the targets, and only 78% of regular businesses.
Table 16: The percentage of businesses who set production targets and have staff members aware of those targets
Type of Employee | Type of Business | Businesses that set production targets (%) | Businesses with employees aware of production targets (%) |
Manager | High Growth | 90 | 86 |
Regular | 86 | 86 | |
Other Staff | High Growth | 90 | 83 |
Regular | 86 | 78 |
5.3 Training Days
The MES also focussed on the amount of training days that a business provided for both managers and non-manager employees. We observed a significant drop in the rate of high growth of businesses that trained their employees for less than a day per year, of 3.5%.
12.2% of businesses that stated that they spent more than 10 days training employees were high growth.
While the extremes of this question show quite a large variation from the average high growth rate, for businesses that spend anywhere between one to ten days training, a roughly average number were high growth. Businesses that invested in over ten days of staff training in 2016, had a higher than average rate of high growth business. We also observed that businesses that invested in less than one full day of training in 2016 had a lower than average rate of high growth business.
Figure 20: Variance from average high growth rate based on staff training days
5.4 Performance Management
Two questions related to employee performance. The first focussed on performance management reviews, and how many members of staff were subject to reviews. When considering the managers of a company, the largest rate of high growth businesses occurs when “about half” of managers undergo a performance review. As the proportion of managers who undergo a review increases, the rate of high growth businesses returns to close to the average rate (10.4%).
This indicates that while there is a benefit to businesses if they give about half of managers performance reviews, enforcing reviews upon every member of management could negatively affect the business and its chances of being high growth.
Figure 21: Variance from average high growth for the proportion of managers under review
The second question regarded how soon a business manages any under-performance of non-managers. The rate of high growth businesses that stated they had no under-performance was 5.9%. Businesses that identified and dealt with under-performance generally had a higher than average rate of high growth businesses, similarly for organisations that addressed under-performance within six months.
The findings for this question may not be as reliable as the others, as around 5,000 of the 9,000 responses were for “within 6 months”. We can still infer some meaning from the findings of this question but may not want to rely on it as much as the other questions.
Figure 22: the rate of high growth businesses, based on management of under-performance
5.5 Performance Bonuses
High growth businesses were more likely to distribute performance bonuses to managers and other members of staff. We also observed a difference in the reasons for awarding bonuses, indicating high growth businesses may have more structured employment practices. 66% of high growth businesses gave out performance bonuses based on meeting a production target compared to 57% of regular businesses, and this pattern was repeated for other members of staff. However, analysing the individual responses of the question showed no difference in the type of target needed to be met for a bonus to be awarded between high growth and regular businesses.
Figure 23: Percentage of businesses who distribute performance bonus based on a target
5.6 Basis for Promotion
Analysis of the reasons that companies gave for promoting staff showed that businesses that engage in positive promotion practices were more likely to have been high growth. For businesses that did not promote any staff, around 6% were high growth. Organisations that promoted staff based mostly on performance had the largest rate of high growth businesses.
This group also included some “other factors” into the process. These factors include restructuring needs and length of service. This indicates that including factors such as restructuring in the promotion process increases the chance of a period of high growth. Conversely, businesses that promoted their employees solely on performance had an about average chance of having a period of high growth.
Figure 24: High growth rates based on promotion practices in a business for non-managers
6. Conclusions
We observe a high rate of high growth companies when we merge datasets with web data from Glass AI. This itself indicates that high growth companies are more likely to have an online presence. Modelling high growth companies using traditional structured business data from the interdepartmental business register (IDBR) results in poor results, with little improvement in classification over random choice. When combining with web data from Glass AI we see minimal improvement, but we do see inputs from the web data contributing to the main features. The main features we see contributing to the models are concerned with company networks, with the organisation and people mentions in or out contributing to the top five. The Glass AI sector code – which could reflect industry sectors not defined in Standard Industrial Classification (SIC) 2007 code – did not appear as a main characteristic in the models used. The merged dataset was small and should be expanded to show a larger sample of UK businesses.
Web text, such as organisation description, is self-generated and self-promoting. Businesses can also start to copy the language used by other companies producing similar and limited topics. However, when analysing the free text used by businesses in their websites we see that high growth businesses are more likely to use terms like “management” and “teams” rather than more specific terms such as “tax”, “law” and “manufacturing”. This could indicate that high growth businesses are concerned more with processes and people rather than specific tools. However, this is a small dataset and needs further investigation into how different sectors behave. Different sector based terms were starting to appear as topics, but more data is required to perform a robust text analysis.
A drawback of the analysis presented here is that the Glass AI data represents one reading of websites’ data on a certain date. This is different to high growth assessment which is over a period of years and IDBR data that is updated annually. The date of reading the websites did not fall into the time span used for determining the high growth flag. By its very nature web-based content is very dynamic and is regularly updated, whilst some is very static and rarely changed. It is challenging to reconcile these different time scales and points and this will affect the ability of the data to be a reliable indicator of a business during the high growth period.
Geographically we see the major proportion of businesses (27%) based in the South East, and the highest rate of high growth businesses in London (21%). This was also reflected when analysing high growth company distribution by district and travel to work areas. A wide distribution of both standard and high growth companies is observed across the UK, but using the rural urban indicator, high growth companies are more likely to be found in major conurbations rather than cities, towns and in the fringes of towns. Using the Ordnance Survey (OS) retail cluster data for six districts of the UK we observe that the high growth companies are more likely to be located in a retail cluster. This rate is particularly the case for the large conurbations investigated (Birmingham and Glasgow).
Due to the relatively small size of the MES merged with the high growth dataset, caution should be observed when concluding that any of the individual management practices explored has a significant effect on the likelihood that a business will enter a period of high growth. However for each of the questions in the MES we observe that there is a small increase in the rate of high growth companies where positive business practices have been implemented. These increases were in the range of 2-4%. Whilst these results do not show a great impact on the rate of high growth businesses, our findings do show similar results to those found by the original MES survey report. The MES survey report identified practices such as Training, Recruitment and Promotion as having an effect on the overall productivity of a business. We had intended to include the MES features in the GlassAI models but unfortunately the size of the dataset from merging all sources was too small. If the dataset could be expanded then this multivariate analysis should be performed to control for the correlations between management practices.
The understanding of what makes a company high growth is varied and complex. Our analysis has confirmed existing research that it’s difficult to predict high growth firms, and that both clustering effects and being well networked is weakly associated to high growth firms. We have, nevertheless, uncovered some interesting relationships which might bear further investigation. Using new data sources such as Glass AI web data and new geographical measures do not give us a step change in this knowledge but do allow a small insight into the characteristics of these businesses. Given further data these insights could be developed further to help tailor targeting and policy to help business that could potentially be high growth.
7. Future Work
There are a number of topics and areas of interest that arise from this work. These include:
- further investigation into different business sectors with both prediction modelling and with NLP – the topic analysis is of particular interest
- with more and matched companies, it would be useful to understand the rural urban classification of each retail cluster; it would also be of interest to use an indicator of the type and size of retail cluster – for example, if the cluster is predominately shopping, entertainment or restaurant – this may influence the location of high growth businesses.
8. Acknowledgements
The authors wish to thank the Department for Business, Energy and Industrial Strategy (BEIS), Ordnance Survey (OS) and Glass AI who have agreed to collaborate with the Campus on this project. Without these collaborations, discussions and the datasets they have provided this work could not have been carried out. The Campus also thanks the Office for National Statistics (ONS) Centre for Subnational Analysis for its work with OS to generate the retail cluster data.
9. References
Anyadike-Danes, M., Bonner, K., Hart, M., & Mason, C. (2009). Measuring Business Growth: High Growth firms and their contribution to employment in the UK. London: NESTA.
Bravo-Biosca, Albert;. (2011). A look at business growth and contraction in Europe. NESTA.
Chawla, N. V., Bowyer, K. W., Hall, L. O., & Kegelmeyer, W. P. (2002). SMOTE: Synthetic Minority Over-sampling Technique. Journal of Artificial Intelligence Research 16 , 321-357.
Department for Business Enterprise and Regulatory Reform. (2008). High Growth firms in the UK: Lessons from an analysis of comparative UK performance. London.
Dobbs, M., & Hamilton, R. T. (2007). Small business growth: recent evidence and new directions. International Journal of Entrepreneurial Behavior & Research, 13(5), 296-322.
Fernandez, A., Garcia, S., Herrera, F., & Chawla, N. V. (2018). SMOTE for Learning from Imbalanced Data: Progress andChallenges, Marking the 15-year Anniversary. Journal of Artificial Intelligence Research 61, 863-905.
Gilbert, B. A., Audretsch, D. B., & McDougall-Covin, P. P. (2008). Clusters knowledge spillovers and new venture performance: An empirical examination. Journal of Business Venturing, 23(4), 405-422.
Gilbert, B. A., McDougall, P. P., & Audretsch, D. B. (2006). New Venture Growth: A Review and Extension. Journal of Management, 32(6), 926-650.
Glass AI. (2018). Glass.AI. Retrieved from Glass.AI: https://glass.ai/
Henrekson, M., & Johansson, D. (2010). Gazelles as job creators: a survey and interpretation of the evidence. Small Business Economics, 35(2), 227-244.
Lechner, C., & Dowling, M. (2003). Firm networks: external relationships as sources for the growth and competitiveness of entrepreneurial firms. Entrepreneurship & Regional Development, 15(1), 1-26.
Nathan, M., Rosso, A., Gatten, T., Majmudar, P., & Mitchell, A. (2013). MEASURING THE UK’S DIGITAL ECONOMY WITH BIG DATA. NIESR.
Paatero, P., & Tapper, U. (1994). Positive matrix factorization: A non-negative factor model with optimal utilization of error estimates of data values. Environmetrics., 111-126.
Porter, M. E. (1998). Competitive Strategy: Techniques for Analyzing Industries and Competitors. New York: Free Press.
Bloom and Van Reenen, 2010. Why Do Management Practices Differ
across Firms and Countries? Journal of Economic Perspectives—Volume 24, Number 1—Winter 2010—Pages 203–224
10. Appendices
10.1 Web reading principles
The Campus have completed a National Statistician’s Data Ethics Advisory Committee self-assessment. Details on Office for National Statistics’ (ONS’) web scraping policy is available. Glass AI (2018) have also supplied the following information concerning how they read open web data:
We follow these principles when reading and structuring text from the public open web:
- Seek to minimise burden on company website owners;
- Honour any potential requests made by website owners to refrain from reading their public website;
- Protect all personal data of potential users and will seek ethical advice before publishing any open web data which may identify individuals;
- Abide by all applicable legislation and monitor the evolving legal situation (especially now with GDPR).
More specifically, our intelligent agent has been built as far as possible to mimic the interactions of a human researcher when browsing the open public web. It does not read full sites; it selectively follows links where it considers the relevant content to be; it is rate limited so a single site is consumed more slowly than a human reader and it only reads lightweight html documents to get text content (not the full-page resources a human user with a web browser would download).
10.2 Data merging
The following table shows the number of records, high growth companies and rate of high growth companies at each stage of data merging with the Glass AI data. The Department for Business, Energy and Industrial Strategy (BEIS) data has a proportion of data with a not applicable (NA) for the high growth flag – companies where the high growth flag could not be determined based on the Organisation for Economic Co-operation and Development (OCED) definition. For example, three years’ worth of data history for that company may not be available.
Table 17: Data summary of the number of records in combined datasets
Number of records | Number of High Growth Companies | Rate of High Growth Companies (%) | |
BEIS | 228,078 | 13,138 | 5.8 |
BEIS (NA removed) | 195,016 | 13,138 | 6.7 |
BEIS + IDBR (NaN removed) | 6,592 | 568 | 8.6 |
BEIS + IDBR + Glass AI (NaN removed) | 5,456 | 488 | 8.9 |
BEIS + IDBR + ONS PC | 6,584 | 568 | 8.6 |
10.3 Coding environment, packages and libraries
Code was developed in Python 3 on the ONS Data Access Platform (DAP) using Cloudera Workbench. Data was stored as distributed files (HDFS). Data was loaded and initial data merging and pre-processing performed using PySpark, before converting to Pandas dataframe. This allowed the use of the imblanced_learn package for data balancing and SciKit Learn and XGBoost packages for machine learning models. Natural Language Processing (NLP) topic analysis was performed using Gensim.
Table 18: Overview of libraries and packages used in the project
Library | Description |
PySpark | Python API for Spark, cluster computing framework |
Pandas | Data analysis and manipulation tool |
Imbalanced_learn | Re-sampling techniques or imbalanced datasets |
SciKit-Learn | Machine learning tools for data mining and data analysis |
XGBoost | Optimized distributed gradient boosting library |
Gensim | Vector space modelling and topic modelling toolkit |
10.4 Classifcation models
Table 19: Summary of the classification models and packages used
Model | Package | |
1 | Logistic regression | SciKit learn |
2 | Linear SVC | SciKit learn |
3 | Gradient Boosted Classifier (GBC) | SciKit learn |
4 | Random Forest | SciKit learn |
5 | X Gradient Boost Classifier (XGB) | XGBoost |
10.5 Imbalanced datasets
A dataset is balanced if the classification categories are approximately equally represented. Almost all real-world datasets are imbalanced, comprising of predominantly “normal” examples with only a small percentage of “abnormal” examples. It is these minority classes that are often of most interest, for example, detecting instances such as fraud or rare cancers.
Machine learning algorithms can have difficulty learning when one class dominates the other. They can learn to class all inputs as the majority class and still achieve high scores. The evaluation of algorithm performance using predictive accuracy alone in the case of imbalanced datasets is not appropriate. Often applications require a high rate of detection of the minority class and allows a small error rate in the majority class, often viewed in a confusion matrix. The receiver operating characteristic (ROC) curve is a standard technique for summarising classification performance over a range of trade-off between true positive (TP) and false positive (FP) error rates. The area under the curve (RUC) and ROC convex hull are traditional performance metrics for a ROC curve. It is often necessary to address the imbalance in the dataset to achieve the appropriate performance from a model.
There are a number of approaches to addressing class imbalance and increase sensitivity to the minority class:
- synthesis of new minority class instances
- over-sampling of minority class
- under-sampling of majority class
- adjust the cost function to make misclassification of the minority instances more important that misclassification of majority instances
The number of observations in the dataset is small and reducing it further was avoided in order to maximise the data used for training models. Synthesising new points and over-sampling of the minority class were chosen as the most appropriate techniques.
10.5.1 Techniques for minority synthesis
Synthetic minority over sampling – SMOTE – synthesises new minority instances between existing (real) minority instances. The technique was proposed in 2002 and is now an established method, with over 85 extensions of the basic method reported in specialised literature. It is available in several commercial and open source software packages. A way to visualise how the basic concept works is to imagine drawing a line between two existing instances. SMOTE then creates new synthetic instances somewhere on these lines.
In Figure 25, we start with an imbalance of four blue against 16 orange instances. After synthesising it is now 10 blue against 16 orange instances, with the blue instances dominating within the ranges typical for the blue values. This is a main aspect of the technique – over-sampling with replacement focused data in very specific regions in the decision region for the minority class. It does not replicate data in the general region of the minority instances, but on the exact locations. As such it can cause models to overfit to the data. In SMOTE, synthetic data is used rather than replacement. The minority classes are oversampled by taking each minority class sample and introducing synthetic examples along the segments joining any or all of the k minority class nearest neighbours. This forces the decision region of the minority class to be more general, leading to better generalising decision trees. Considering a sample , a new sample will be generated considering its k nearest neighbours. The steps are
- take the difference between the feature vector sample under consideration and its nearest neighbour χzi
- multiple the difference by a random number γ between 0 and 1 and add this to the sample vector to generate a new sample χnew
χnew = χi + γ(χzi – χi)
Figure 25: Schematic overview of how SMOTE creates new data points based on existing data
Initially there is an imbalance of four blue against 16 orange instances (left). Lines are drawn between the four minority data points (middle) and extra points are added at a random point along these lines (right). After synthesising it is now 10 blue against 16 orange instances, with the blue instances dominating within the data range typical for the original blue values.
A variation of this approach is adaptive synthetic (ADASYN) sampling method. This operates in a similar way as regular SMOTE, except the number of samples generated for each χi is proportional to the number of samples that are not from the same class of χi in a given neighbourhood. SMOTE will connect inliers and outliers in the data, while ADASYN can focus solely on outliers. This can sometimes lead to suboptimal decision functions. To help address this SMOTE has different implementation options to generate samples (in fact, over 85 different extensions to the regular SMOTE methods have been proposed). For example, in the SciKit Learn imbalanced-learn library these methods – SVM, Borderline1 and Borederline2 – focus on samples near the border of the decision function and will generate samples in the opposite direction of the nearest neighbour class.